Driving on the Edge: How Edge Analytics Will Enable an Autonomous Driving Future
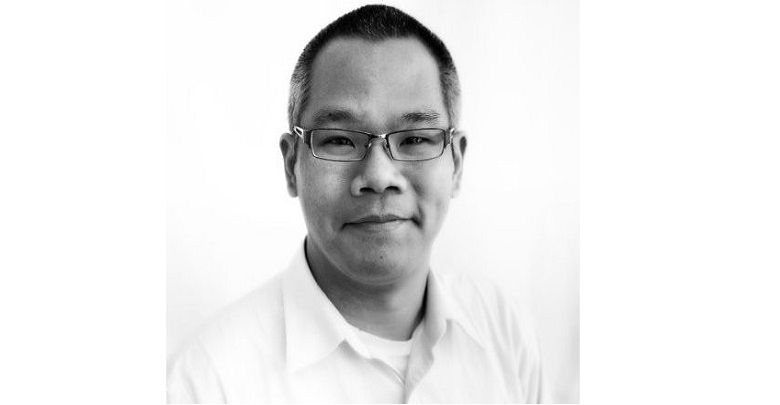
Most consumers are aware that their smartphones are generating mountains of usage data every day. The more apps they download, the more browsing they do, the more purchases they make, the more data their phone creates to enhance their user experiences.
As cars become more connected and sophisticated, they too will generate vast amounts of usage data. Like smartphones, consumers will become more and more reliant on the data generated by their cars—and data analytics—to keep them safe, drive efficiently, and extend their digital lifestyles into the cockpit. To put it in perspective, today’s connected luxury vehicles generate 25 gigabytes of data per day. By comparison, fully autonomous vehicles in the future are estimated to generate a whopping 4 terabytes of data per day.
A looming problem for automakers will be how to minimize the expense associated with transmitting all this data while optimizing vehicle response times for safety critical advanced driver assistance systems (ADAS) and autonomous driving capabilities and simultaneously keeping the vehicle’s price down for consumers. One solution lies in automotive “edge analytics.
Edge analytics is an approach to data collection and analysis where data is accessed and analyzed at the point of data capture—in this case within the connected vehicle itself. Managing data and analytics in this way minimizes the amount of data transmission between the vehicle and the cloud, with the goal of enabling as much advanced data-driven functionality as possible while keeping vehicles independent from the cloud in the event there is no network connection available.
In situations where the automobile can be fully connected, edge analytics still has the benefit of reducing transfer and latency times for vehicle systems that are data and analytics dependent, allowing them to process information and react in real-time. This balance between autonomy and connectivity could be imagined as a scenario where analytic modules are updated over-the- air for specific use cases. For example, the vehicle could learn or be tuned to perform best for the geographic area where it spends most of its time. If it drives beyond those borders, it can download an updated intelligence module. When the automotive manufacturer discovers a new algorithm that improves the vehicle’s image recognition during nighttime driving or in a blizzard, it can send that new analytic module to the automobile.
Edge analytics will also be fundamental for enabling connected vehicles to optimally operate in vehicle-to-vehicle (V2V) and vehicle-to-infrastructure (V2I) scenarios where on-board analytics translates into faster and better interactions with the outside environment – which will be particularly important for fully autonomous vehicles operating without human drivers. Like how edge analytics improves performance and reduces cost by pushing decisions closer to the vehicle, pushing localized data that will be consumed by the automobile to the edge of the network also improves response times and reduces overall transmission costs. Hyper-localized
data can then be instantly accessed by edge analytic modules, allowing a vehicle to quickly respond to an accident ahead or prepare for dangerous road conditions due to black ice that is detected by the vehicle in front.
If automakers want to remain competitive while providing drivers with the safest and best in-car experiences, they must include edge analytics in their connected vehicle technology portfolios. This is the best way to deliver upgradeable advanced functionality to their automobiles while also improving performance and reducing costs for the consumer.