Multi-sensor Analytics based advanced fleet management for Shared Mobility
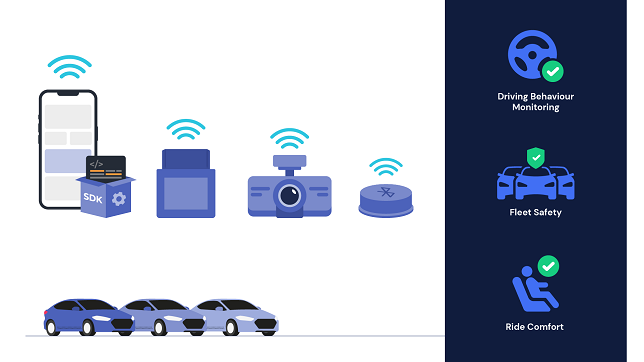
Use of Telematics for Fleet Tracking and other Fleet Management functions has become increasingly prevalent. Even in emerging markets, the attach rate of Telematics in fleets is growing aided by enhanced awareness, regulation and maturity of affordable Telematics service offerings.
This article is not about repeating the key use cases of Telematics in Fleet Management, which are fairly well established by now. However, this article is about what we view as the next wave of technology adoption for fleet management, especially for fleets involved in shared mobility.
By shared mobility, we define any shared use of a vehicle – car, motorcycle, scooter, bicycle, or other travel mode such as a bus. Typically, it’s a short-term access for travel as needed, either as a co-passenger or as a self-drive mode.
From GPS Telematics to a Multi-Sensor Approach
Conventional Telematics use cases in Fleets have centred around a GPS Tracking Device (Telematics Device) which has a built-in GPS and Cellular chipsets to obtain and transmit Location information of a vehicle to a central server in near real time. Using a GPS Tracking device for Track and Trace and other Location related features is still the highest priority use case for fleets.
Over the years, these Telematics devices have become more capable with additional on-board sensors and interfaces. These include Inertial Motion Sensors (IMUs) for measuring vehicle acceleration for Driving Behaviour monitoring and OBD/CAN interface for getting access to ECU for Fuel/Vehicle health monitoring. Optionally. Additional I/Os that connect to Relays for remote Immobilization and Fuel Sensors provide additional sensing and actuating features.
Telematics for Fleet Management is moving towards a Multi-sensor architecture where multiple devices (including GPS, Camera, Smartphone and Smart sensors) using a combination of At-the-Edge and Cloud Computing deliver the key Use Cases.
The centrepiece of this Telematics scenario is the Telematics Device that is the primary data gathering device to collect and transmit data to the Cloud based Telematics Server. Cloud Telematics Server ingests data from all the connected devices and performs real time and historical time series data analytics to enable various use cases for Locational Intelligence, Driving Behaviour or Fleet Health Monitoring.
We see this Telematics Architecture evolving is in two ways, especially when its related to Fleet Management for Shared Mobility:
- Use of multiple sensors and sources of data apart from the Telematics Device to make use of different type of data – this includes Video, Additional external Sensors and access to proprietary OEM’s ECU data
- Use of At-the-edge computing to derive actionable insights within the Vehicle in combination with the current In-cloud data processing approach
We explain the above with the example of a representative EV Shared Mobility Service that provides chauffeured EV Cabs for in-city mobility. Such a Fleet needs Fleet management technology to ensure the following key use cases:
- Location Tracking – This includes Location Intelligence and Notifications
- Safety of Drivers and Co-passengers and reduce risk of any accidents
- Ensure comfortable and pleasant ride experience for passengers
- Ensure Fleet Uptime, Utilization and prevent unnecessary breakdowns
- Being an EV Fleet, have updated information on Battery SoC/SoH and Charging status
Since the Shared Mobility is operating in a highly competitive environment, it needs to be best in class across all the above to maintain competitive advantage.
In such a scenario, conventional Telematics Devices can only fulfil a part of the Telematics Value Proposition. Instead, a multi-sensor approach that make use of other Data sources and Devices is the preferred approach (See Figure 1).
The multi-sensor approach includes:
- Driver’s Smartphone Sensors that capture location and additional driving behaviour of the Cab Driver
- Dashboard camera feed to monitor the front traffic and in-cab view for enhanced safety and monitoring risky driving behaviour
- Additional sensors such as BLE Smart Beacons placed at different locations in the cab to capture data for riding comfort and in-cab temperature
- If available, data feed from OEM fitted Telematics Device from OEM’s Data Center to get additional insights on Vehicle condition
- Charging data feed from Charging infrastructure used by the Fleet to get valid and real time information about the charging status
Notice that many of these additional data sources are either a different type of data or a different method of data collection as compared to the conventional Telematics Device.
Also, as compared the conventional approach where data is captured and transmitted to the cloud in conventional Telematics, some of this new Data being captured is voluminous and thus needs to be processed At-the-Edge in order to generate usable insights in an efficient and timely manner.
Let’s take a deeper dive into some of these new sensor data technologies
Driver Smartphone sensors for Safe Driving
Typical Telematics Devices rely on GPS based Speed as the primary data source for measuring Driving behaviour. While several Telematics Devices do come with accelerometers to measure vehicle acceleration, the lack of processing capability on the device generally hampers the accuracy of Safe Driving behaviour alerts such as Hard Acceleration or Sudden Braking. Also, some of the more risky Driving Behaviour behaviours such as Distracted Driving (picking up the phone for calling or text) are not captured.
That’s where Smartphone Sensor based Telematics can come in handy. Smartphone Telematics App automatically collect Driver’s smartphone sensor data (including accelerometer and gyroscope) when the Driver is on duty and the cab is moving. High frequency Sensor data is captured and processed on the Phone itself enabling highly accurate Safe Driving Behaviour determination including Distracted Driving. (see Figure 2)
Typically available as an Mobile SDK, Smartphone Telematics can be seamlessly incorporated into the Driver App being used by the Driver for the normal workflow of the Shared mobility operation.
Wireless Sensors for Ride Comfort
Apart from ensuring Ride Safety, it’s important for a Cab Fleet to ensure a comfortable and pleasant ride. Here, we see use of Smart sensors that measure Temperature and Acceleration being experienced by the passengers in the cab. Located near passenger seats, these low cost, low power, BLE sensors are battery powered and transmit IMU and temperature sensor data wirelessly to the Driver Smartphone App (See Figure 3).
These sensors can also detect an accident like event and generate a real time alert to the server for emergency response.
Again, this data is processed at the edge on the Driver’s Smartphone during the ride. Using AI based algorithms on this sensor data, one can monitor the actual jerks and bumps being experienced by the passenger. This along with the in-cab temperature monitoring can ensure Ride comfort.
Video Telematics for Enhanced Safety
In conventional Telematics Data Processing Systems, the data from Telematics device is analyzed for speed and acceleration information of the vehicle with varying level of accuracy depending upon the accuracy of the sensors in the Telematics device. This information gives an absolute measure of driving behaviour irrespective of the traffic context in which the driving is happening.
Some of the limitations of pure telematics-based driving behaviour monitoring approach include:
- Over-speeding information does not capture the front traffic condition i.e. whether the over-speeding occurred on an empty road, road with moderate or dense traffic. Since the accident risk of over-speeding on an empty road is lower than accident risk of over-speeding on even a moderate traffic road, severity of over-speeding event should depend upon the road traffic condition.
- Sudden Braking event when the vehicle is closely followed by another vehicle is more severe as compared to a sudden braking event on an empty road. In another scenario, a Sudden Braking event when the vehicle approaches very close to nearest vehicle or pedestrian in front is a risky (or a near accident miss) event.
- A quick-lane change event when the vehicle is entering a lane that has a closely following vehicle is more severe as compared to a quick lane change event into an empty lane
In each of the above cases, pure telematics-based driving behaviour monitoring systems cannot provide adequate visual context to rate the severity of the rash driving incident
These limitations can be overcome by use of video feed from Dashcam fitted in the vehicle or captured from the docked smartphone camera of the cab driver. A Machine vision-based Video data processing system continuously captures and processes the video data to identify potentially dangerous driving events. Such continuous video data processing is however computationally intensive as it involves Deep Learning algorithms to run on one or more video streams at real time speed. This requires systems with high processing and memory requirements, thus increasing the cost of deployment.
An alternative approach is to process the video data only for the time in the vicinity of a driving behaviour event detected by the Telematics data processing system (See Figure 4). Also, the system need not process the video stream in real time but process the frames over a few seconds after the event has occurred. This dramatically decreases the video processing load on the system enabling the video processing to be implement at the Edge in relatively moderate capacity devices like smartphones and vehicle head units.
OEM and Charging Infra Data Feed
Most modern vehicles have OEM fitted Telematics and other ECU data capturing units. Shared Mobility Fleets should advantage of that data by partnering with OEMs and integrating their Telematics Server with the data feed from OEM’s data center. These can provide additional CAN sensor data typically not easily accessible to after-market Telematics Device.
This OEM data feed can supplement that Telematics data collected by the after-market Telematics device can be used for advanced Health Condition Monitoring of various modules of the Vehicle, Predictive/Preventive Maintenance and Fuel/Energy efficiency monitoring.
For EV’s, such feed can provide additional proprietary data related EV Battery SoC/SoH, Charging status and other vital parameters for monitoring the battery status and overall health of the Battery, BMS and related modules. (see Figure 5)
Additional Feed from external Charging Infrastructure can be used to check the location and availability of charging slot in real time, cross-check the charging status, charging current and ensure right charging practices.
Multi-Sensor Telematics as source of competitive advantage
The above example of an EV shared mobility illustrates the power of multi-sensor Telematics approach in enabling higher value Fleet Management functions across safety, efficiency and customer satisfaction standpoints. Such approach can be equally applied for other forms of Shared mobility. Starting with the desired Use cases, one can architect the right combination of sensor data capturing and processing paradigm to serve the Use cases while ensuring Return on Investment on the resources spent on Technology. With such an approach, Telematics transitions from a necessity and cost centre to a source of true competitive advantage for Shared Mobility Fleets.
Author:
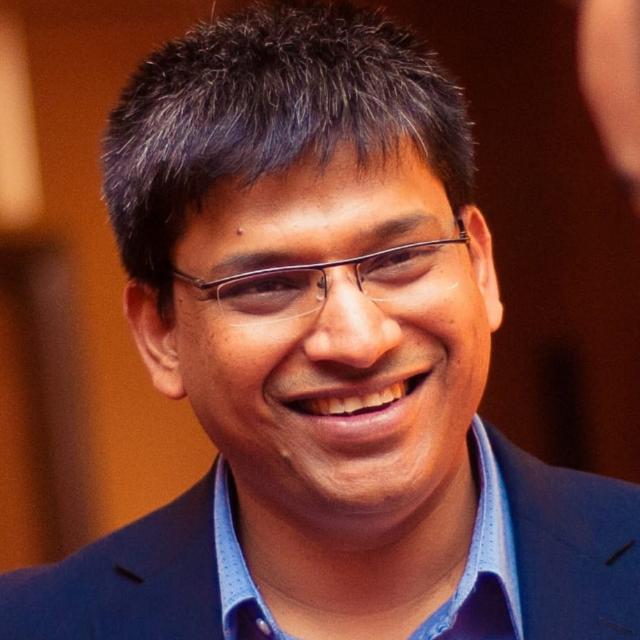
Kamal Aggarwal
Co-founder and CEO
SenSight Technologies Private Limited
Kamal Aggarwal is Co-founder and CEO of SenSight Technologies, a start-up working at the intersection of Automotive IoT and Big Data. SenSight’s AutoWiz Connected Vehicle Data Analytics Platform powers use cases for players in the Automotive, Insurance and Shared Mobility domain. Kamal has 20+ years of experience in Strategy, Marketing and Product Management for high tech ventures.
Published in Telematics Wire