How the rise of vehicle electronics and connectivity will impact the future of automotive manufacturing
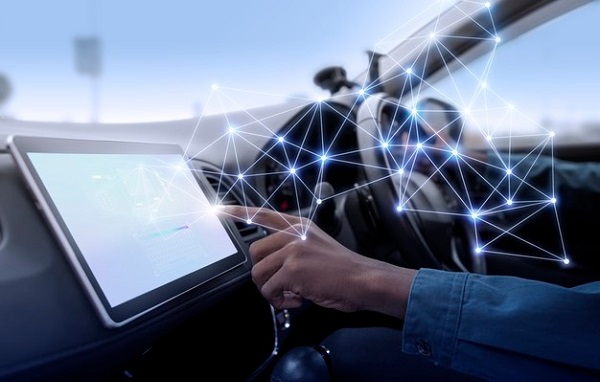
To define a future-proof infrastructure architecture for Smart Factory, we must start by understanding the future of automotive manufacturing. The future car, and its ecosystem & lifecycle, will define its infrastructure requirements.
The dramatic growth of electronics content in vehicles in recent years is driving major changes in the automotive industry. While Advanced Driver Assistance Systems and Autonomous Driving (ADAS/AD) development have been a major focus, electrification of the vehicle drivetrain has recently become very significant as companies seek greener alternatives to the internal combustion engine. At the same time, vehicle connectivity, 5G availability are both seeing unprecedented growth. All of these factors are driving semiconductor content within each car higher at a time when the pandemic has taught us that traditional just-in-time manufacturing methodologies don’t necessarily work for semiconductors.
What’s exciting though is that on-board electronics and 24×7 connectivity bring with them new opportunities – from manufacturing quality improvement to enhanced customer experiences and even new streams of revenue – some of which haven’t been discovered yet. The one common thread is data. Data is king. The more access vendors have to real-time data, whether from in the smart factory or from the customer’s connected car, the more opportunity to benefit. But only if companies are prepared to leverage it.
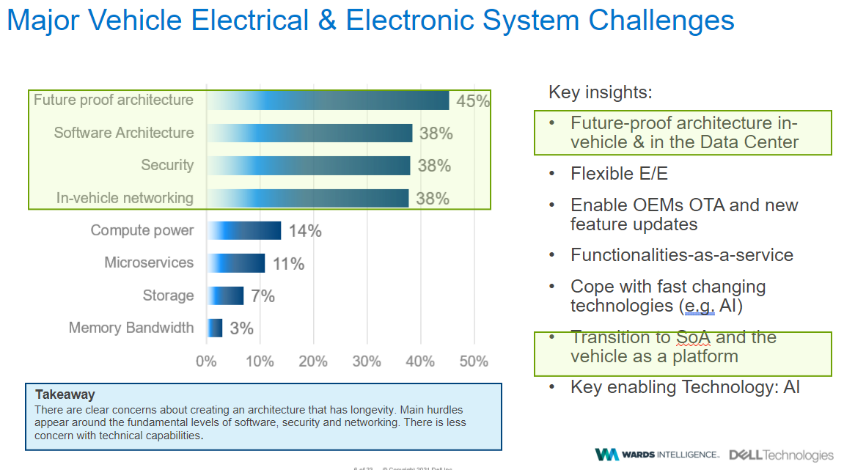
Dell Technologies has been working extensively with Original Equipment Manufacturers (OEMs) and Tier 1 Automotive suppliers for many years and recently sponsored a Ward’s Automotive survey focused on future vehicle design trends with emphasis on the impact on infrastructure requirements. Wards surveyed over 300 global automotive professionals from OEMs; Tier 1 and Tier 2 suppliers; a mix of manufacturing operations; quality; supply chain; and purchasing professionals to help us define the future requirements of vehicles. Once we understand what the future car looks like and what kind of ecosystem is needed to build it, we can then define the future infrastructure requirements for Smart Factories.
One of the first areas that the survey focused on, for obvious reasons, was electrical and electronic system challenges. From the survey it became clear that there is a great desire for creation of a future-proof architecture for in-car electronics. This is supported by the fact that companies are moving toward a service-oriented architecture that is software defined. Interestingly, the survey respondents also placed a high priority on a future proof architecture for their data center.
To understand why companies are moving toward a software-defined car we only need to look at some of the recent luxury cars on the road today. As was noted by Bosch, it is not uncommon for a luxury car to have over 100 electronic control units (ECUs). Each ECU has its own software, and the total software content in many cars is already over tens of millions of lines of code. They estimate that a fully autonomous car could increase the software content to nearly 500 million lines of code. A look at how ADAS solutions are being built today makes this easy to understand.
The Society of Automotive Engineers (SAE) has divided the level of autonomy of a car into 6 levels, with SAE Level 0 being non-automated and SAE level 5 being fully automated. Most advanced cars on the road today are SAE level 2. SAE Level 3 cars are just now starting to appear. If we take a closer look at these cars, what we see under the hood (pun intended) are multiple ADAS subsystems, (blind-spot detection, pedestrian detection, adaptive cruise control, etc.) that have been interconnected to create an advanced capability. Self-parking is one obvious example that combines multiple subsystems. The problem here is that typically each subsystem comes with its own ECU and software. As you interconnect them, another ECU is added, and software must then be written to perform “sensor fusion”, which enables multiple sensors to work together. If we look at the sensors, many have their own ECUs as well. Further complicating the situation is the fact that there are multiple bus standards being used within the typical car. Add it all up and you have a very complex, safety critical system that can be difficult to debug and maintain.
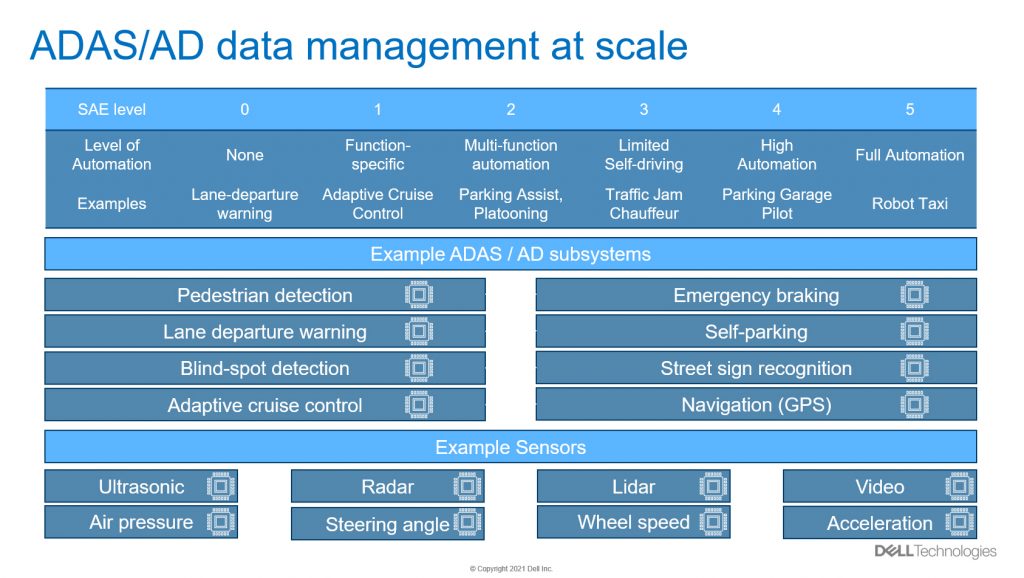
For SAE level 5, we must capture multiple exabytes of data, and will require hundreds of CPU cores and thousands of GPUs to conduct testing. And all that data still must be stored for decades.
So this brings us to the future-proof in-vehicle architecture. By moving to a service-oriented architecture, where there is only one ECU (or a few), maintaining the vehicle becomes significantly easier. In essence the vehicle of the future will be like a cell phone – where all the hardware is preinstalled and all you need to do is download an app to enable it. If you think about it, this isn’t really new. Many cars today come pre-equipped with satellite radio and all you need to do is purchase a subscription (and maybe download some updates). The car of the future will be very similar, except you’d be buying and downloading advanced features like emergency braking, blind spot monitoring, or even full autonomy. What’s exciting for the OEM is that this can create new revenue streams – like renting features. For the customer, this is also exciting. It’s easy to imagine the customer that doesn’t drive very often, and therefore doesn’t need full autonomy (or maybe couldn’t afford to buy it up-front). But that customer could be interested in buying it for a weekend to drive to/from the mountains. That represents incremental revenue for the OEM, and a benefit for the customer as well.
Note that 24×7 connectivity really expands the opportunities for the OEMs to enhance services. Telemetry data from the vehicle can be analyzed in real-time to predict part failures and direct the customer to a dealership before something catastrophic happens. Likewise, with historical driver data, they can selectively offer extended maintenance contracts. Additionally, telemetry data can be transmitted back into the Smart Factory. Predictive quality, which uses real-time data streaming along with historical data to predict when a part will fail during manufacture, can be extended to predict, during its manufacture, that it will fail after it has shipped and is in actual use. This reduces warranty costs while improving quality. All this is exciting, yet the most significant aspect of connectivity is the opportunity for new revenue streams in that OEMs can collect by offering new data-driven services. Maintenance of the car is one obvious example. Another could be pushing restaurant coupons based on driver history and real-time location. The truth is that we don’t know what the future will bring, but if we provide the data, someone will figure out how to profit from it.
And that brings us to the demand for the future-proof data center. Today, companies that are experienced in ADAS / AD development know that careful planning is needed to manage the enormous amounts of sensor data that must be collected for AI algorithm design and validation. Our experience has shown that a typical SAE level 2 vehicle requires on the order 5-10 petabytes (PB). SAE level 3 grows that requirement to 50-100+ PBs. Many have predicted that SAE level 5 storage requirements will be measured in exabytes. Already companies must think carefully about how they build their data centers for ADAS/AD. Now we also have to think carefully about the factory.
In the Smart Factory, we have features like predictive quality and predictive maintenance. In the typical factory today, parts run through various stages of production, with quality checks at the end of each stage. In spite of our best efforts, some parts that pass all up-stream inspections will still fail at the very end. This occurs when there are less-obvious defects in the parts that inspections miss – often because they simply don’t know how, where, or why to look for them. This is where the power of AI comes into play.
To deploy AI we first must collect lots of data across the factory floor – all the typical data we would normally use for inspections (Ex: machined part tolerances, chip diagnostics), plus less obvious data (relative humidity, air temperature, power line noise, floor and/or machine vibration, acoustical data, solder source, part images, etc). Parts failures are also tracked. The data is then analyzed, and AI algorithms used to predict when parts will fail. For example, it could be determined surface-mount chips will develop solder cracks whenever the solder temperature is at the highest temperature band, the air pressure is low, and a nearby drill press is generating excessive floor vibration. With this knowledge, these parts can be rejected (and corrective measures taken) before they are fully assembled. Predictive maintenance is very similar – except here the algorithms are analyzing the manufacturing floor equipment and for the above example, would predict that the drill bit in the drill press needs replaced before excessive vibrations cause part failures and a temporary factory disruption.
Smart Factory is very similar to ADAS/AD development in that both are AI-dependent and rely on large amounts of data to power the process of training and continuously refining AI algorithms. Though Smart Factories don’t generate data at the magnitude of ADAS/AD, they can still generate petabytes of data when imaging data is collected. Surface-mount chip installation is one example where imaging is used to track precise chip alignment, which directly impacts quality.
So factory data centers are already expected to grow in size. Now add hundreds of thousands of connected vehicles, each on the road streaming data back to the factory across 5G, 24×7 and it’s obvious that total data is going to grow. For the Smart Factory, that telemetry can be used to extend predictive quality to the vehicle. Again, storage requirements grow. So it’s no surprise that the survey indicated that a future-proof data center is also important. No one wants to invest in massive amounts of infrastructure today, and then realize later that it doesn’t scale – forcing a fork-lift upgrade a few years later. This is why the industry is looking for an infrastructure architecture that is future-proof – that can adapt and grow with the data.
The good news is that there are solutions available that are architected for precisely this sort of situation. Dell’s Autonomous Drive Ecosystem, for example, is used by our ADAS/AD development customers. These customers already see exponential growth of PBs of data, and through experience, know that a well architected infrastructure it’s not simply storage at scale. The future architecture must deliver consistent, high performance to feed hundreds of GPUs and thousands of CPUs concurrently for algorithm development and validation – even as their ADAS/AD infrastructure scales out over the years. The same infrastructure must include hardware and software that both enables data storage and management. Sensor data, for example, must be indexed – it must be searchable by data scientists. Such an AI-centric infrastructure architecture is easily adaptable to the future Smart Factory, which itself is AI-centric.
It is worth noting that though infrastructure capable of capturing and maintaining data for AI development is essential, it is useless if your data scientists do not have the tools to use it. This was reflected in the same Ward’s survey, which noted that data scientists spend far too much time preparing data for AI development vs. using data for AI development. This was one reason why Dell Technologies introduced an ecosystem of partners that collectively, provide an open software reference architecture for AI development. With the right partners, companies can automate their data management and tool flow orchestration – freeing Data Scientists to focus their time on what they do best – AI Algorithm design.
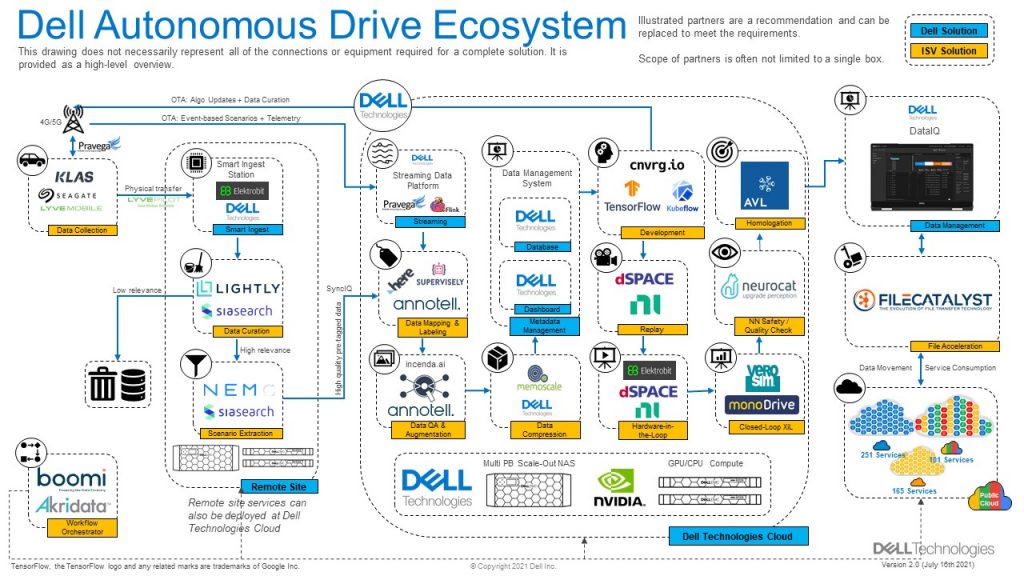
In the future automotive Smart Factory, the focus will be on connectivity, where the Smart Factory itself is going to extend to the customers; enabling predictive quality and predictive maintenance to leverage post-production data. The future Smart Factory will also extend backwards to include suppliers. The supply chain will itself become connected and stay connected for the life of the vehicle.
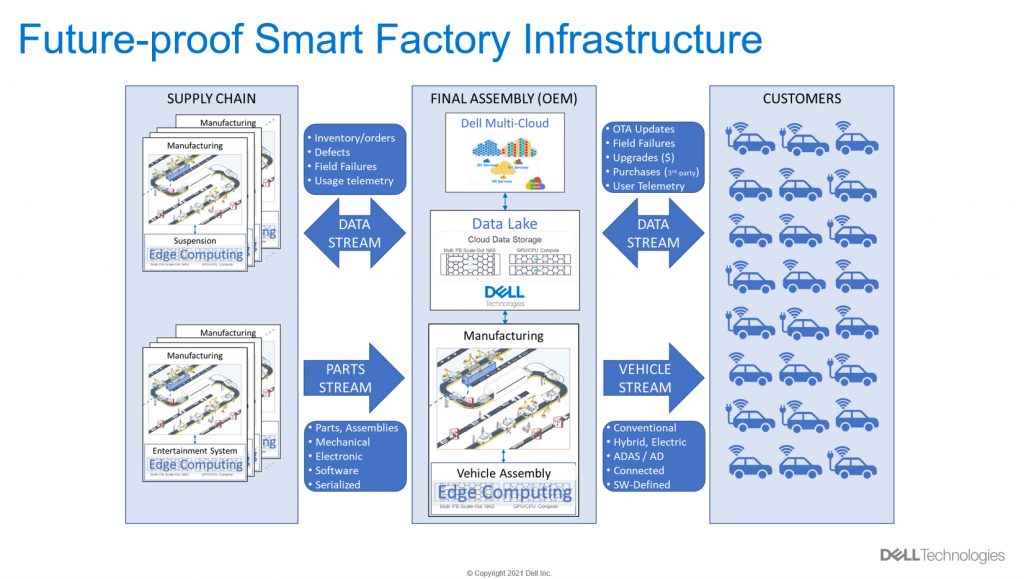
The Dell Technologies Automotive Hub is updated frequently with new events and content related to ADAS/AD, Smart Factory, and the Dell Autonomous Drive ecosystem. For more information on Dell Technologies solutions for automotive, please visit DellTechnologies.com/Automotive.
Author:
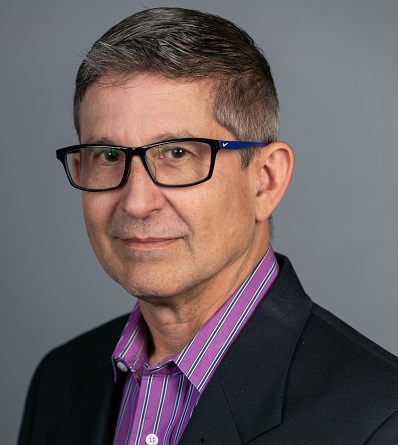
Lawrence Vivolo
Sr. Business Development Manager
Dell Technologies
Lawrence Vivolo brings more than 30 years in high-technology design, marketing and business expertise to his role as Senior Business Development Manager for Automotive and Semiconductor Design and Manufacturing for Dell Technologies, Unstructured Data Solutions division. He holds a BSEE from California Polytechnic State University, San Luis Obispo, and an MBA from Santa Clara University, Santa Clara, California.
Published in Telematics Wire