Automotive Testing and Simulation with AI insights
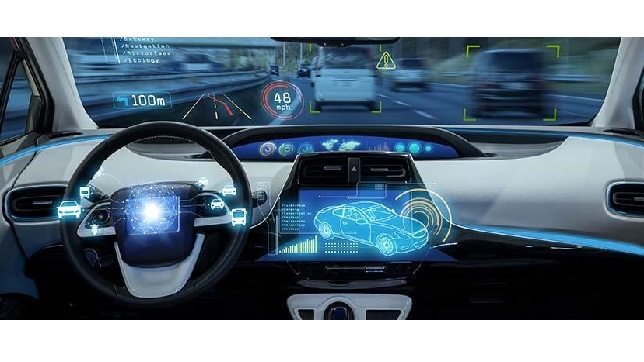
Challenges for testing and the simulation of autonomous and connected vehicles
The expense of developing new hardware and software to achieve a viable, autonomous and connected vehicle system is considerable. Using intelligent simulation applications, you can identify possible issues early, and fix them faster. This will help you avoid costly mistakes and ensure your ideas are validated before building an expensive solution.
By capturing data collected from geospatial sources, a high fidelity ‘digital twin’ of your simulated world can be created. Also, by injecting consumer behavior data, the movement of vehicles and pedestrians through this world can be predicted using deep learning algorithms. Once a living digital twin is established, a developer is free to use design thinking principles to refine their autonomous and connected system. The simulation will define how quickly and safely the vehicles could be trained in a risk-free virtual world, and could highlight likely outcomes when the system is taken out into the real world.
With intelligent simulation technology a developer can perform multiple parameter sweeps to test traffic flow, scheduled maintenance, challenging weather conditions, accidents, connectivity issues, vehicle range, refuel or recharge rates, CO2 emissions and even cost expenditure per trip.
What is ADAS and how can it bring safer roads?
Advanced driver assistant systems (ADAS) is being used since decades in order to ensure safer driving to help drivers by warning about potential dangers and reduce the roads hazards and incidents. It can also ensure driver inattentiveness or common mistakes can be avoided.
ADAS functions are already mandatory on new vehicles in some regions around the world, one of the example being the anti-lock braking system/autonomous braking system becoming mandatory across Europe since 2004.
A report by ‘Wholesale Insurance Provider Swiss Re’ indicates that due to the advanced ADAS system the road accidents reduced by 45.5% on motorways and 27.5% reduction on other roads in the UK.
With the future inclining towards autonomous driving cars we would need connected cars and with advanced communication of Vehicle to Vehicle, Vehicle to Infrastructure and Vehicle to Everything communications.
Use of AI for vehicle simulation
“With the self-driving cars, there will be AI and data driven strategies at every step to drive simulation and smart connected systems”
For an autonomous vehicle to be a SAE level 5 vehicle and reach ‘Vision Zero’ in theory they should be safer than human operators with no place for error.
Deep Learning algorithms and data analytics will serve as a backbone for assisting drivers ahead of time for any dangers and a safe road experience.
AI with deep learning algorithms can carry out complex data intensive calculations to read surrounding environment information including moving objects, pedestrians, detecting traffic lights, movement of other vehicles, detecting type of vehicles, extracting road context information and communicate successfully in real time to the connected infrastructure.
Simulation and Testing steps
- For connected cars, we need to check Connectivity for vehicle to infrastructure and vehicle to vehicle communication. With the upcoming 5G, as latency for data transmission shall reduce, it can bring in processing of data collecting and processing much faster.
- The second part is, critical scene understanding as these are an inclusive part of simulation – vehicles, pedestrians, cyclists, animals and texture-map surface elements and this is exactly what Waymo has been doing simulation by using the camera images collected by its self-driving cars.
- A creation of 3D environment to mimic the data driven system with 3D geometry, semantics, appearance of objects within the scene and also testing the weather conditions like snow, hailstorms, fog, heavy rains, sunlight and the simulation will analyze the scene and have decision making based on different angles and varying distances.
- As the scene changes, the AI model should be able to learn and adapt to the updated environment and recreate additional data sets.
- Once these elements fall in place we now need to test the cars from a virtual world to real road with safety.
EYE ON IT – Current Industry Standards
Some of the deep learning algorithms the companies are looking are Convolutional Neural Networks (CNN), Bayesian regression, Decision forest regression, K Nearest Neighbor, Principal Component Analysis, Histograms of oriented gradients, K-means, multi-class Neural Network, Gradient-Boosting and AdaBoosting. With the complexity of intensive computation for object detection, classification, combination of Regression, clustering, Pattern recognition, decision making algorithms are used. But more complex the algorithm, we need to be mindful that it does consume a lot of fuel once it is tested on the road due to the computational power.
Types of Simulators
Driver in Loop (DIL) Simulators, one such application would be in gaming applications and installations in theme parks and trade shows. This can be extended to Hexapod motion bases which are human factor DIL, need to measure human driver behaviors and are an expensive investment. The Dynamic vehicle simulation systems are the newest of simulators to mimic the real world driving scenarios and can also help manufacturers.
Emergency Medical Services (EMS) and Simulation
The Goal of EMS is to increase the patient survival and reduce the response time to reach the scene of the incident. To improve the response time to reach the incident, dispatch and relocation planning needs to be done.
The simulation system to test the EMS coverage to find the ambulance availability, travel time, anticipated demand that can then help to find shortest time to reach the incident area.
For emergency calls, ambulances are deployed across different locations already so they can reach faster at site, simulation programs in the 3D environment can help to test real world scenarios of multiple ambulances being tested based on variant calls from diverse areas.
AI can be used to calculate and predict ambulance location and relocation problems, ambulance dispatching, staff scheduling, demand forecasting, simulation and queuing for evaluating performance of EMS system, and decision support systems with Geographic Information Systems (GIS).
The simulation systems also need to consider the increase in ambulance numbers, different shifts, addition of ambulance stations, increase in number of calls, ambulance specialization, hospital selection, inter-hospital transport, changes to relocation and rerouting policies, fleet composition.
With the combination of AI and simulation systems, with the virtual world testing involved, we can ensure better response time, cost-effective and scalable AI based EMS in the future.
References:
https://venturebeat.com/2020/05/20/waymo-is-using-ai-to-simulate-autonomous-vehicle-camera-data/
Authors and Co-Authors
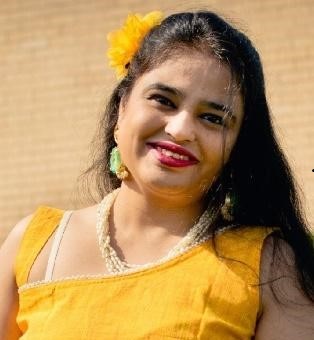
Jaisal Surana is a business and data analyst with the Telecom domain with in-depth knowledge on product lines, architecture, billing experience for 14 years in IT with Tech Mahindra. Part of the MKAI steering committee and AI Ethics Forum, her interest as a tech enthusiast allows exploring all NextGen technologies
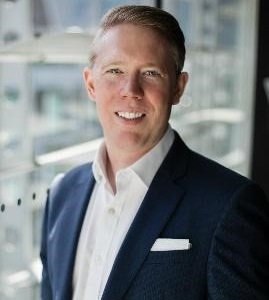
Richard is an Artificial Intelligence (AI) Influencer, Speaker and Podcast Host. He is the Founder of NeuralPath.io, an AI Advisory Practice, Chair of MKAI and Host of the Boundless Podcast: Designing Our Digital Future. He is a graduate of the MIT AI Strategy Course and a Wiley published author on the Future of AI.
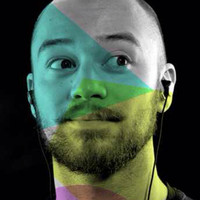
Steve is the Product Designer (UI/UX) for Immense Simulations. He has been a design professional for 13 years and has provided UX and design expertise to a diverse range of industries from manufacturing to E-commerce. Currently, he is helping Immense disrupt the simulation industry by designing the next generation of simulation SAAS (software as a service) tools.
Published in Telematics Wire