Building safe, secure and reliable autonomous vehicles
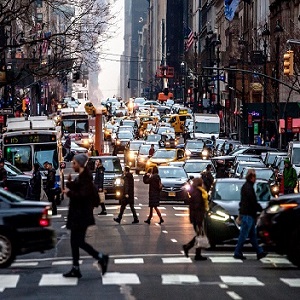
Nearly 15 years ago, autonomous driving was a science fiction. Today, the world is fast moving towards this goal with many OEMs already in advanced stages of testing their prototypes. Autonomy is being considered and built into everything that in the past, relied on the humans to make decisions. We see this trend catching up from flying machines to ground vehicles to everything in between, including ships and vessels.
It all started with automation. Humans are creative creatures that do not like repetitive and monotonous tasks. We find every opportunity to automate something that is not mentally stimulating. This curious extension to automation today has grown into a full-fledged autonomous phenomenon replacing humans from most unlikely places.
In our experience, every time a machine replaced a human – that job never came back to humans. This is because machines do the job much better than the humans they replace. Machines have stretched the boundaries in every sense – be it speed, precision, accuracy, reliability or safety. So far, in all the mechanization scenarios, the informed decision making has been with humans.
Can you trust an autonomous vehicle?
When machines are becoming autonomous, it means that they are designed to make decisions on their own, independent of human intervention. This brings a new set of challenges. Trust is an invisible thread that underlines all human interactions. Today, when we take a Taxi, we trust the driver knows his job and that he or she will safely drive us to our destination. When there is no driver in your Taxi, who do you place your trust on? How do you know that the machine is trained to handle all likely scenarios? How do you trust that the machine will not compromise your safety? How do you trust that the Taxi you are in, is not under the control of some unscrupulous elements that don’t mean well to you?
In 2018, SAE International had conducted a survey for Ansys, where nearly 80% of the participants said that they don’t feel safe in a fully autonomous vehicle. Building trust and confidence in autonomous vehicles is a key challenge for people to fully adopt the technology.
Reliability of autonomous vehicles
In an autonomous vehicle, the primary decision making is done by Artificial Intelligence (AI) based software. A specialized branch of AI called machine learning (ML) / deep learning (DL) algorithms make decisions that are based on our understanding of how neural networks of the human brain works. Our eyes provide visual sensory inputs based on which the brain creates a perspective that provides image recognition and creates depth perception. The human brain can process much additional information and use its learning from real-world experiences (intuition) – to make decisions even when it encounters a scenario that it never dealt with before. This is where things become challenging for artificial intelligence.
The AI based perception algorithm learns from manually labeled training data from scenarios. Higher training data sets can improve the ability of perception software. However, it is impossible to cover every conceivable scenario. We can group scenarios into known and unknown classes. In each class, the vehicle behaviour may be classified as safe or unsafe. The most dangerous combination involves unknown and unsafe scenarios, also called Black Swans. Training the perception software to cover this class of scenarios is not possible as we do not know them.
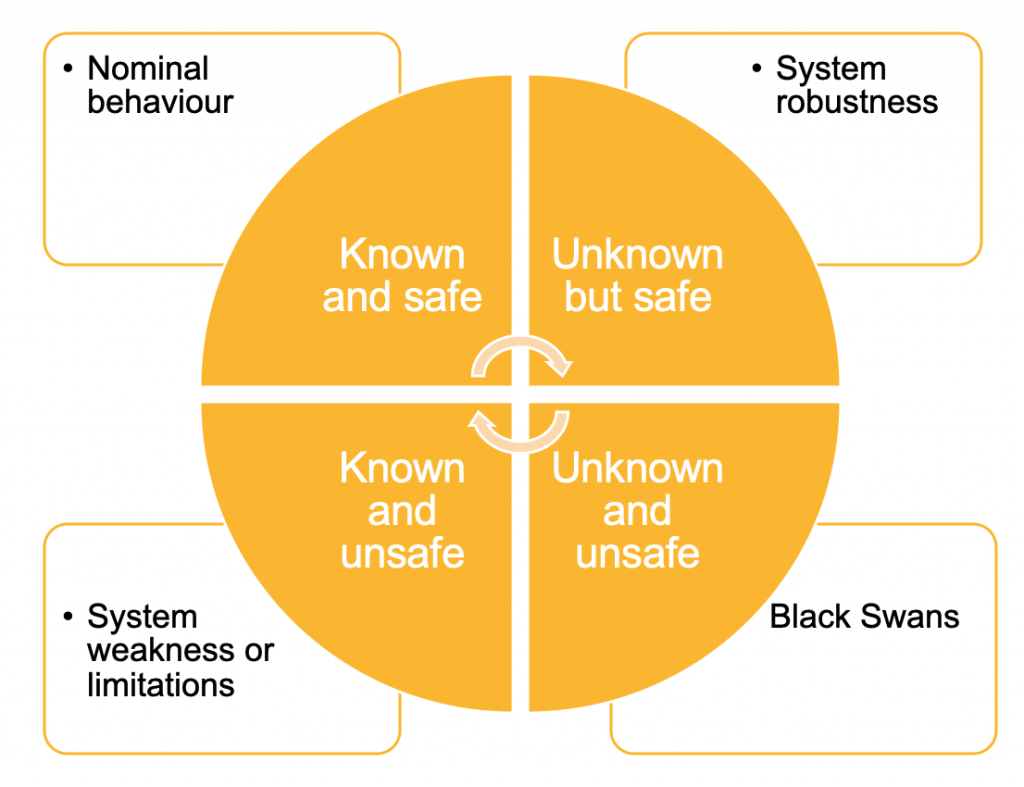
When we look at safe and unsafe situations for known scenarios, we can quickly realize that any known safe situation can turn into an unsafe, due to unfavorable environmental conditions. A scenario which seems to be playing out perfectly normal under a regular situation may fail due to an incremental change in weather condition. A flickering road lamp or a lightning may cause the perception algorithm to read the road signs incorrectly. An AI software that can detect adult humans with high degree of confidence may fail to detect a child or a person on wheelchair. There might be occasional wildlife crossing the road. It is totally unacceptable for an autonomous car to not detect these vulnerable road users. These are typically called edge cases or corner cases – that an AI software should manage safely.
The manual methods used by perception system engineers is to label objects in videos and then program their autonomous perception systems to respond correctly – are time consuming and expensive. Given their time and cost prohibitive nature, it simply doesn’t make sense to rely on these manual methods to identify every conceivable edge case. Unique solutions that are designed with the practical needs of perception system developers can help improve the robustness of the perception algorithms.
Safety is non-negotiable
Safety is a very important topic when it comes to automotive, especially with autonomous vehicles. ADAS and AD are heavily dependent on numerous ECUs to provide driving and safety functions. ISO 26262 provides detailed guidelines to understand various risks due to malfunctions and recommendations to mitigate the same to an acceptable level. The ISO 26262 standard has been the current state of the art which is widely practiced in the industry to ensure safety.
While functional safety covers risks associated with malfunctions due to failures in electronics, defects in software or manufacturing process, Autonomous Vehicles also have to deal with risks due to insufficiency of autonomous functions, known as safety of the intended functionality or SOTIF. This covers areas such as limitations of sensors, insufficiently defined algorithms or reasonable misuse.
Sensor limitations may include situations where a camera sensor reaches saturation due to extremely bright light (which may be due to an oncoming vehicle) or a patch of dirt stuck at the lens affecting camera functions. Insufficiency of algorithm could limit the ability of perception software to identify a specific kind of road sign or object, in certain geographical locations. A driver may not respond to a system event within specified time which is typical misuse to be expected of from humans. An autonomous vehicle has to consider all these (and more) possibilities so that functions are executed as intended, without causing safety issues. Autonomous driving requires a huge amount of road testing by practically driving these cars in all traffic situations on public roads. How many miles would autonomous vehicles have to be driven to demonstrate their failure rate to a particular degree of precision? As per a research article published by RAND Corporation, “this number is approximately 8.8 billion miles. With a fleet of 100 autonomous vehicles being test-driven 24 hours a day, 365 days a year at an average speed of 25 miles per hour, this would take about 400 years”
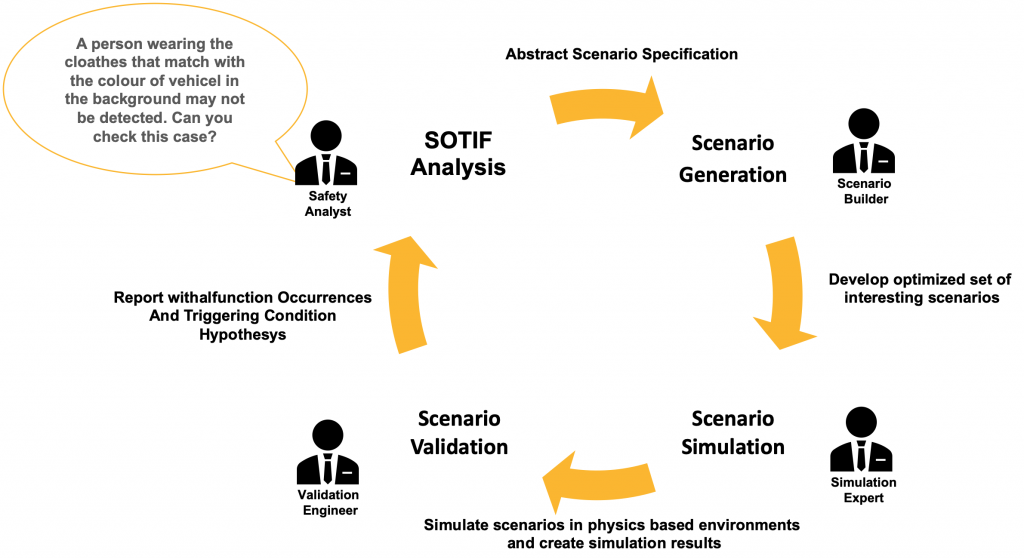
It is not possible to achieve this target with road testing alone. An alternate approach is to simulate majority of the scenarios including what-if scenarios, in a physics based virtual environment. Safety analysist can start with the analysis of SOTIF triggering conditions, which can be used to develop a variety of optimized, parametric scenarios that can be simulated in a virtual environment and edge cases can be identified in synthetic video. Virtual simulations provide best of both worlds – to provide an extensive coverage of scenarios covering billions of miles, without causing risk to the lives of people and the physics behind these simulations ensures the results are accurate and as close to the real world as possible.
Cyber-physical systems are always targeted for security exploitation
Autonomous cars are cyber physical systems that are practically computers on wheels. These vehicles rely extensively on connected infrastructure to ensure safety and deliver superior user experience. However, these functions can potentially offer opportunities for an intruder to hack into vehicle systems. Successful attacks might cause data leak causing privacy issues and progressively take control over the vehicle functions. This may result in denial of service where a vehicle may become unavailable to service the needs of its legal owner. Financial risk including the damage to properties is a likely scenario. Deliberate acts of misleading the perception algorithm can turn out to be the most dangerous hack. Most importantly, loss of security also means loss of safety. A compromised autonomous vehicle can pose serious risk to lives of people and instill fear thus breaking the confidence for adoption.
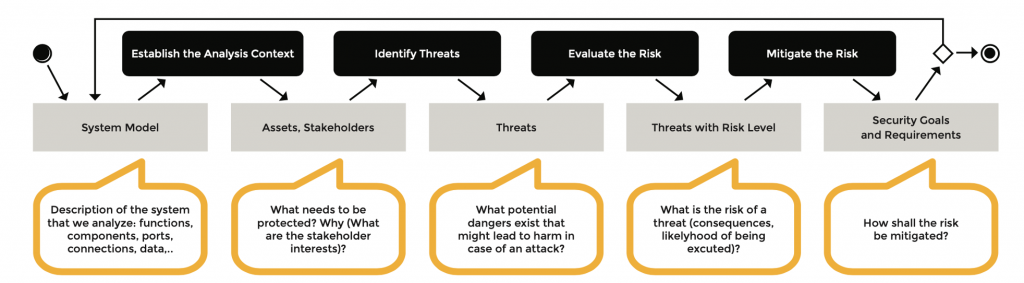
The autonomous vehicles must be systematically analyzed for every possible security threat by identifying vulnerable assets and performing threat analysis and risk assessment. Any unreasonable risk must be systematically resolved. Certain functions that are too risky may have to be discontinued. However, it must be noted that improving the security comes at significant cost. Apart from redesigned safety functions, hardware with higher performance (to deal with encryption / decryption), additional security specific functions, and the vehicles must be continuously monitored for security exploitation throughout their lifetime, across the markets.
Conclusion:
The real driving force behind the autonomous vehicles is reliability, safety and security. As discussed before, it is important to ensure that autonomous decisions taken by the system are most appropriate, for the situation. Be it ensuring the correct behavior of sensors, robustness of perception software or deterministic controls coupled with driving situation simulations – physics-based simulation is the closest we can get to real road testing.
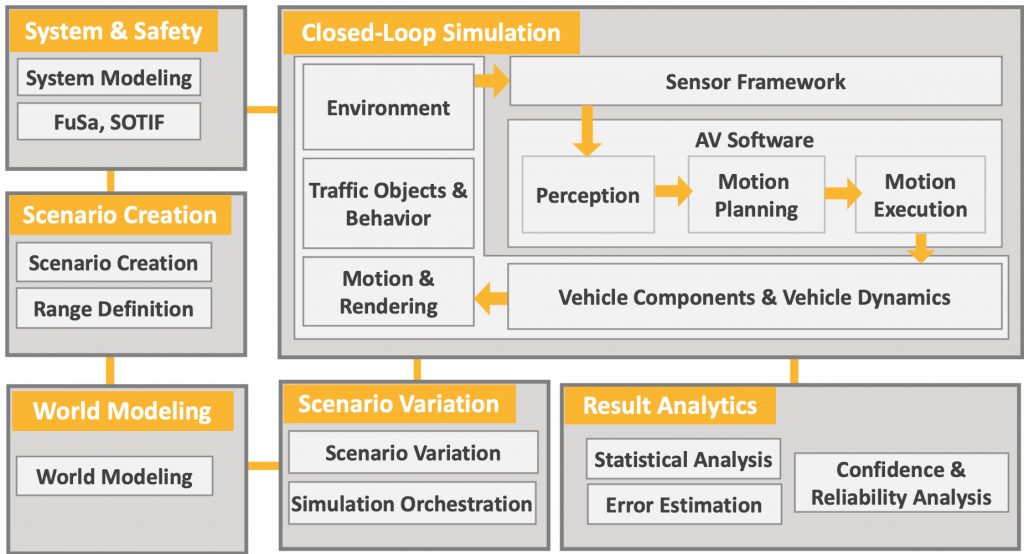
Developing a fully autonomous vehicle that never causes risk to humans is a far-fetched idea. The collective effort of the industry is to make autonomous cars much more safe, secure and reliable than humans. An integrated approach that complements virtual simulations with safety and security perspective can help address most concerns and get us closer to realizing trustworthy autonomous vehicles.
References:
- https://www.rand.org/pubs/research_reports/RR1478.html
- https://www.nhtsa.gov/technology-innovation/automated-vehicles-safety
- https://www.iso.org/standard/68385.html
- https://ieeexplore.ieee.org/document/8468619
- https://www.ansys.com/about-ansys/advantage-magazine
- https://www.ansys.com/resource-library/white-paper/resolving-visual-edge-cases-safer-autonomous-vehicles
- https://www.ansys.com/solutions/technology-trends/autonomous-engineering/sae-survey
- https://www.ansys.com/about-ansys/advantage-magazine/volume-xii-issue-1-2018/drive-safely
Author:
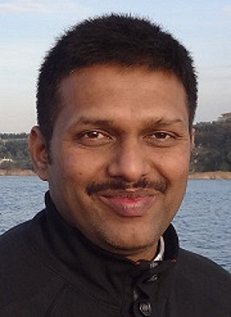
Raghavendra Bhat is Technical Manager for India, South East Asia for SBU at ANSYS. Earlier, he worked for Esterel, IBM and Telelogic. He work experience includes- SDLC including requirements management, software configuration management and model based design & development. He has M.Tech(Software Systems) from Birla Institute of Technology and Science, BE(E&C) from VTU and a Diploma in Electronics.
Published in Telematics Wire
One Comment