Neuromorphic/Event-Based Vision Systems: A Key for Autonomous Future
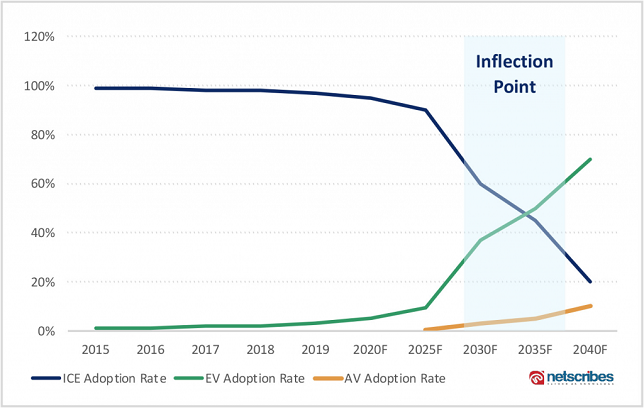
We are getting closer to a future with driverless cars, and the promise of autonomous vehicles is more exciting than ever before. Robo-taxis will be the first fully AV to be commercially available by the end of 2025, with autonomous personal cars following suit by 2030. In the years to come, the fate of driverless technology will depend on how well it can replace human vision and make decisions just as humans do.
As of today, vehicles are powered by SAE level-2 and level-3 technology, with OEMs aiming to leapfrog to level-5 autonomy. For the next five years, one of the major focuses will be on perfecting computer vision technology to replace human vision.
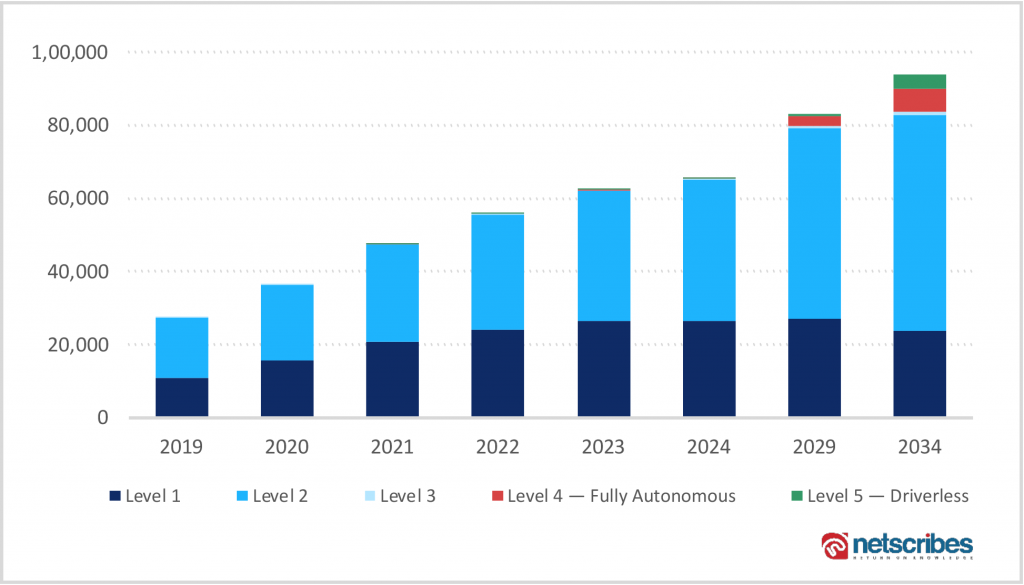
Computer vision has emerged as an integral part of autonomous vehicle technology, unleashing the transformative benefits of the humble and traditional camera system. Today, computer vision systems are based on frame-based acquisition for capturing the motion of an object using several still frames per second (FPS). In such systems, vision sensors collect a large chunk of data corresponding to all the frames captured by the camera. However, a significant portion of the data collected during the frame-based approach is unnecessary and only adds to the overall size and intensity of data transmission and computation. This, in turn, exerts an immense burden on the vehicle architecture. Although these vision systems work well for various image processing use cases, they fall short of specific mission-critical requirements for autonomous vehicles.
Neuromorphic or event-based vision systems are concentrated on emulating the characteristics of the human eye which is essential for an autonomous vehicle. These systems operate on the changes in the brightness level of individual pixels, unlike frame-based architectures where all the pixels in the acquired frame are recorded at the same time. Event-based vision sensors follow a stream of events that encode time, location, and asynchronous changes in the brightness levels of individual pixels to provide real-time output. These systems, therefore, offer significant potential for driving the vision systems in autonomous vehicles as they can enable low latency, HDR object detection, and low memory storage.
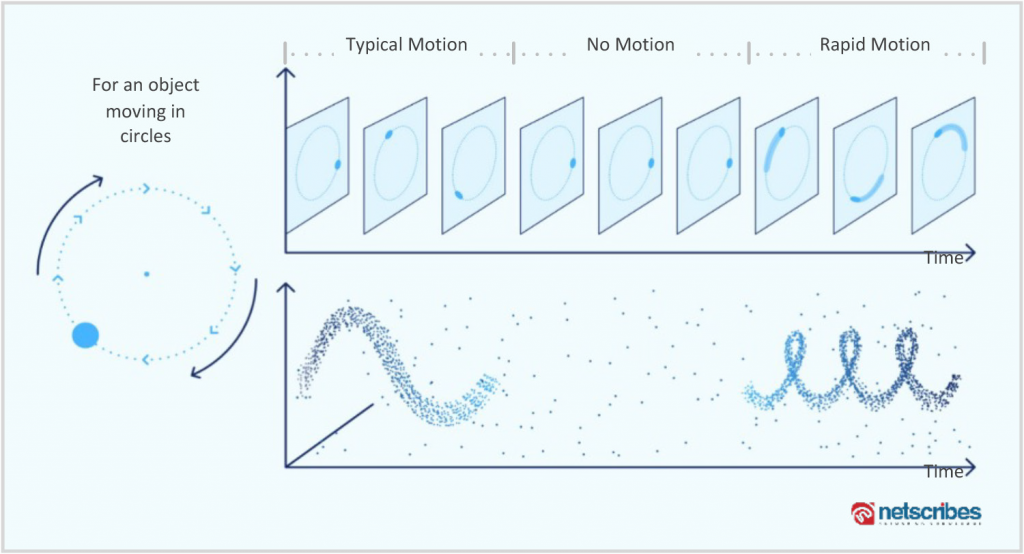
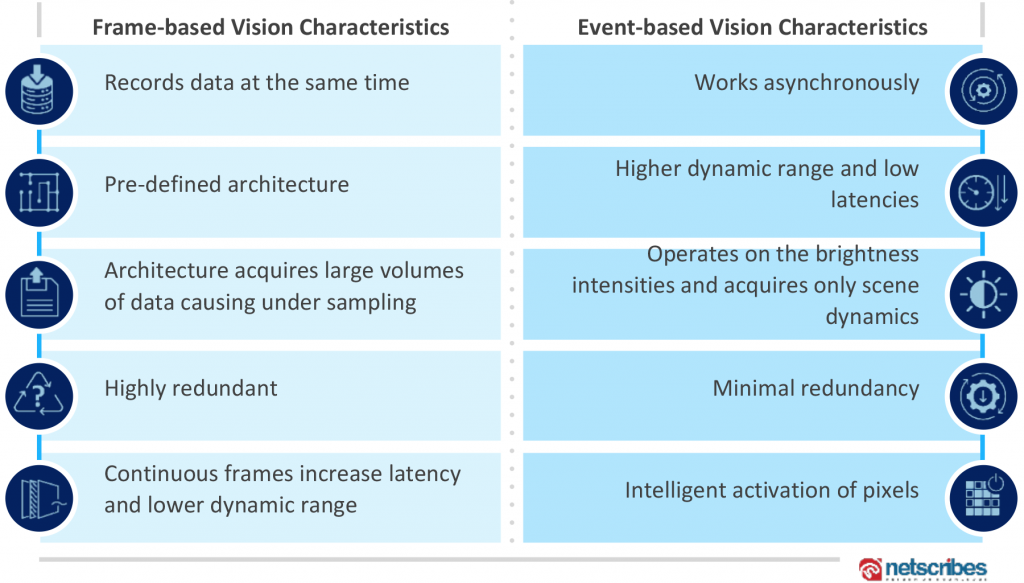
Event-based vision technologies have been in development for over 15 years now. They have been evolving for most of the 2000s and have begun to gain momentum during the current decade.
Applications such as autonomous driving are now looking for a more human-like technology that can effectively replace humans. Hence, event-based vision sensors will be a natural choice of AV OEMs going forward.
– Faizal Shaikh
Key Development Areas in Event-based Vision System:
Accuracy in Optical Flow Calculation: Current optical flow algorithms are complex and computationally intensive. These are used in conventional frame-based cameras. Event-based systems will follow a new optical flow technique that is driven by brightness. Companies such as Samsung and CelePixel are active in developing technologies that follow an on-chip optical flow computation method. This technique uses a built-in processor circuit that measures changes in the intensity at individual pixel levels.
Power Consumption: Depth-mapping computation in event-based cameras is power-intensive as all the pixels need to be illuminated to calculate the time of flight. Furthermore, motion recognition of fast-moving objects consumes a higher amount of power. Generally, low-power, event-based cameras are affected by noise that leads to inaccurate calculations. Thus, Samsung and Qualcomm have been exploring ways to optimize power consumption in even-based sensors using single-photon avalanche diode (SPAD)- based pixels.
Calibration Issues: Event-based cameras have a microsecond-level temporal resolution for detecting millions of events per second. Inaccuracies and interferences in the calibration of event-based cameras need to be addressed for motion sensing and tracking applications. University of Tsinghua’s invention focuses on the spatial calibration method for a hybrid camera system combining event-based cameras, beam splitters, and traditional cameras.
Color Detection: Currently, event-based cameras only detect changes in light intensity and cannot detect colors with the same intensity. Companies are developing color filters to make event-based cameras more color-sensitive. Samsung has been developing an image sensor chip with a color sensor pixel group and a DVS pixel group enabled by a controlled circuit. The configuration of the chip recognizes 2D colored images and reduces power consumption using a power monitoring module.
Frontrunners in the Automotive Event-based Vision Systems:
Mitsubishi (3D Pose Estimation): The OEM has been actively working on 3D pose estimation which is an essential step in localization applications in autonomous vehicles. This system is aimed at developing an edge-based 3D model by matching 2D lines obtained from the scene data with 3D lines of the 3D scene model. In other terms, the focus of this technology is to extract the three important motion parameters (X, Z, and yaw) that help in estimating the DVS trajectory in a dynamic environment. Furthermore, the significance of localization is maintained in this system by using IMUs for obtaining the height, roll, and pitch without compromising on X, Z, and yaw.
Luminar Technologies (LiDAR and 3D Point Cloud): The company has developed a method for correlating DVS pixels of the events with the 3D point cloud of a LiDAR sensor. In this method, DVS is used for selecting the region of interest (ROI) of the scene and sending it directly to the LiDAR beam scanning module, therefore eliminating complexities related to object detection and classification procedures involved in image processing. Consequently, the LiDAR sensor scans only the portions of the scene that are changing in real-time, reducing overall latency.
Volkswagen (Object Detection and Classification): The OEM has developed a neuromorphic vision system for image generation and processing to support advanced driver assistance applications and automated driving functionality. The bio-inspired system includes multiple photoreceptors for generating spike data. This data indicates whether an intensity value measured by that photoreceptor exceeds a threshold. The digital NM engine includes processors running software configured to generate digital neuromorphic output data, which is velocity vector data to determine spatiotemporal patterns for 3D analysis for object detection, classification, and tracking.
Magna (Collision Avoidance): The tier-1 supplier has developed a vision system with a sensing array comprising event-based gray level transition sensitive pixels to capture images of the exterior of a vehicle. The camera combines an infrared, near-infrared, and/or visible wavelength sensitive or IV-pixel imager with the gray level transition sensitive DVS pixel imager. When an object is detected, this technology will alert the driver, while in motion, as well as add an overlay to the image for better display.
Honda Research Institute (Sensing Road Surface): The use of event-based cameras for road surface intelligence can provide information such as the presence of potholes, gravel, oily surfaces, snow, ice, and data related to the lane marking, road grip, geometry, friction, and boundary measurements. Real-time 3D road surface modeling can also help steer transitions from one road surface to the other. The institute has developed a method that uses an event-based camera to monitor the road surface along with a processing unit that can use this signal to generate an activity map to obtain a spectral model of the surface.
Tianjin University (Multi-object Tracking): Another way of using event-based processing on the roads is to track multiple objects at once in real-time. Such situations demand high recognition accuracy and high-speed processing. An autonomous vehicle needs to be continuously aware of the surroundings, should be able to track pedestrians, and needs to have the ability to distinguish between dynamic and static objects on the road. Tianjin University is developing an AER image sensor for real-time multi-object tracking. The method includes data acquisition by the AER image sensor, real-time processing, monitoring light intensity change, and performing output operation when the change reaches a specified threshold. It also includes filtering of background information to reduce data transmission quantity and redundant information.
Samsung (Inaccuracies Introduced by Non-event Pixel Points): The existing camera system solutions face a challenge of non-event pixel points formed due to inconsistent distribution and amount of event pixel points from the right and left DVS cameras. These non-event pixel points do not participate in the process of matching pixel points, consequently leading to erroneous depth information. Samsung has developed a device and method for accurately determining the depth information of the nonevent pixel points based on the location information obtained from multiple neighboring event pixel points in an image. By improving the depth information accuracy of non-event points, the depth information of the overall frame image is improved. In addition, this method eliminates the need for calculating the parallax of the non-event pixel points, thus improving the matching process and increasing efficiency.
Concluding Remarks:
In its early stages, this technology was designed to help the visually impaired. However, over the next 2-3 years, it is estimated to have proof-of-concept for self-driving cars, factory automation, and AR/VR applications. The different benefits of this new concept over the traditional camera system make it suitable for object tracking, pose estimation, 3D reconstruction of scenes, depth estimation, feature tracking, and other perception tasks essential for autonomous mobility.
Automotive companies should consider R&D, either alone or in partnership, related to the deployment of event-based cameras for different applications based on computer vision, gesture recognition (pose estimation, gaze tracking), road analysis, security, always-on operations, ADAS applications, and several other indoor and outdoor scenarios. Automotive manufacturers can also leverage the potential of AI and event-based cameras to develop ultra-low power and low latency applications. Such solutions are less redundant due to the sparse nature of the information encoded.
Additionally, funding projects in the domain can help automotive companies to become a part of the event-based camera ecosystem. Companies such as Bosch, Mini Cooper, and BMW have already become part of projects with research establishments like ULPEC or Neuroscientific System Theory. LiDAR in combination with an event-based camera could be another key area of interest for the advancement of autonomous vehicles. This may help overcome the resolution limitations of traditional cameras. Prophesee is one of the companies that aims to use its event-driven approach to sensors such as LiDARs and RADARs.
Vision systems are getting adopted on a large scale at both the consumer and enterprise level. The event-based approach has the potential to take the vision systems to the next level. It has all the essential qualities to attain the ubiquity expected for the future of autonomous mobility, where neuromorphic sensors will be seamlessly embedded in the fabric of everyday life.
– Siddharth Jaiswal
Authors:
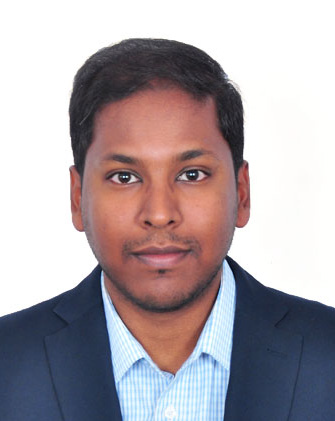
Siddharth Jaiswal
Practice Lead – Automotive
Netscribes
Siddharth has been tracking the automotive industry for the past nine years with a keen interest in the future of mobility, autonomous technology, and vehicle E/E architecture. He leads the automotive practice at Netscribes and works closely with the major automotive companies in solving some of the most complex business challenges.
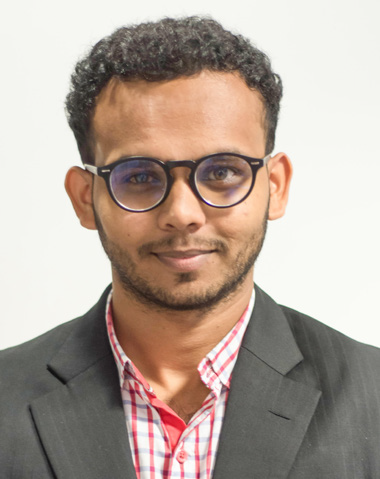
Faizal Shaikh
Manager – Innovation Research
Netscribes
Faizal has six years of experience in delivering digital transformation studies that include emerging technology domains, disruptive trends, and niche growth areas for businesses. Some of his key areas of interest include AI, IoT, 5G, AR/VR, infrastructure technologies, and autonomous/connected vehicles.
Published in Telematics Wire