Simulated world for ADAS/AD development, testing and validation
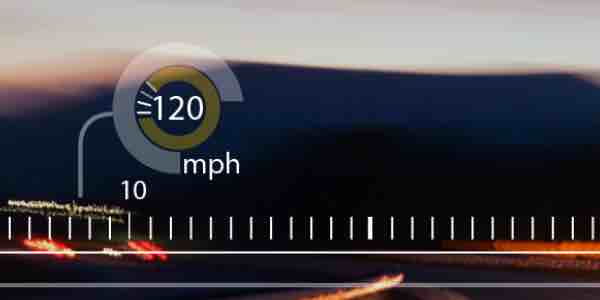
The automotive sector is gearing up for a revolution. Just like the last century saw transition from bullock carts to automobiles, this century is about to witness a transition of a much greater level – the rise of autonomous vehicles. Path-breaking technologies and algorithms have been introduced to tackle this challenge and move towards complete autonomy (Figure 1).
Safety is paramount when developing any vehicle, whether driven by a person or a computer. During the development of autonomous vehicles, the self-driving technologies must be evaluated iteratively in a variety of driving conditions to ensure they are safer than human-driven cars. For deep learning, there is a dire need for enormous amount of data. However, it is estimated that billions of miles must be driven before we can realize the dream of autonomous vehicles. But it is practically impossible to gather these billions of miles in such a short time frame given we are anticipating the revolution in the coming few years. What the world needs is intelligent system that delivers billions of miles with unparalleled cost & time efficiency.
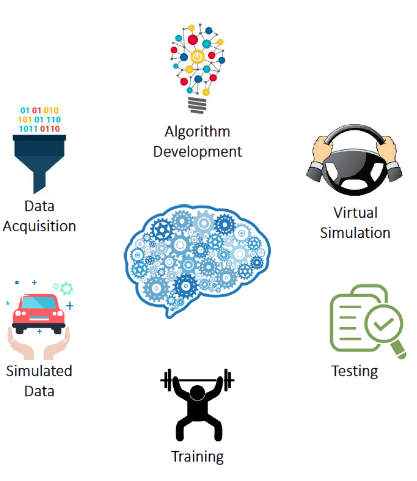
Hence, simulated synthetic datasets are an ideal solution, enabling continuous, fast and unlimited data collection in the digital environment with relatively low operational costs. Self-driving simulations have advantages in mileage data collection efficiency, road condition dataset diversity, and sensor data accuracy. The flexibility and versatility of simulation make it especially valuable.
In particular, simulation can be effective in testing dangerous or uncommon driving conditions. Without simulation, it would be far too dangerous to determine how self-driving vehicles react to certain real scenarios – like a child darting out into the street from behind a parked car, or another vehicle running a red light.
Virtual simulations and synthetic data offer a faster time-to-market as simulations reduce the time required for physical prototyping and real time road testing. It is easier and more economical to test and improve an algorithm in simulation than using a car for live road testing. Being virtual, one can create unlimited amount of data with boundless variations and diversity while keeping low costs. The virtual environment simulation can be used for validation against requirements, sensor fidelity, platform performance, and environmental conditions.
Functionality and Usability for ADAS/AD Development
Virtual simulations and synthetic data generation are boost development of ADAS/AD –
Data Acquisition
Deep Learning or Neural Nets rely heavily on training sets which determine how accurate these models will predict and react to unknown scenarios. Synthetic data provides these training models with large amounts of balanced pre-labelled dataset with sufficient diversity and accuracy – a dream-come-true for deep learning engineers. Photorealistic environments add to these advantages by filling the gap between real and virtual worlds.
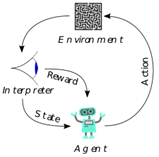
Algorithm Development
Virtual simulation set up allow for faster algorithm development. Model-in-loop and Software-in-loop techniques efficiently bring to surface bugs and logic errors, which would otherwise cause catastrophic implications and increase time-to-market due to physical testing. Reinforcement Learning, as shown in Figure 2, can be ideally visualized and implemented in the virtual set up, again paving the pathway for more realistic algorithms.
Sensor Simulation and Validation
ADAS/AD algorithms are undoubtedly dependent on sensor data. The performance of various sensors (lidars, radars, ultrasonic etc.) under different conditions can be very accurately predicted using high fidelity physics engines in simulations. The time consuming and costly field tests can be substituted with simulations which will provide vital information about sensor operation in real life before actually using it physically.
Components of Synthetic Data and Virtual Simulation Tool
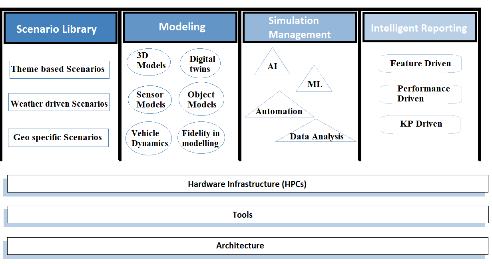
Figure 3 shows the sub systems of this tool. Each sub system is explained further.
Scenario and Environment Design
Scenarios are the first step towards creating real road environment in virtual conditions. This means building catalogues that have millions of scenarios. A library of scenarios is developed considering variations such as the road type, radiant, curvature, junctions, weather conditions, and other parameters. In addition to this, libraries of traffic signs, pedestrians, vehicles, buildings, vegetation, animals etc., need to be created while maintaining variety and diversity.
Modelling
To imitate and simulate real scenarios, an effective modelling of vehicle dynamics and sensors is of utmost importance. The environments and scenarios created should also be as close to real world as possible. While vehicle dynamic models have matured, the maturity of sensor models is evolving. Concepts like digital twins, procedural generation, object modelling, 3D modelling, etc. are being explored. They help in creating a virtual environment as close to the real world. The most important aspect of validation is how close one can make the virtual environment compared to the real- world environment. Machine learning, artificial intelligence, Internet of Things (IoT), and many such technologies need to be integrated to provide a robust and efficient solution for simulation management.
Simulation Management
Scenario libraries and modeling provides the required virtual environment which replicates the real environment to the best of its abilities. However, to make use of this virtual environment, AI tools and simulation is essential. When we talk about AI tools, we are talking about ADAS/AD algorithms, software-in-loop, hardware-in-loop and data analytics. This will enable the user to generate synthetic data required for training as well as test and validate automation algorithms.
Intelligent Reporting
The final step in any process is evaluate that process both quantitatively and qualitatively. This can be done in the following ways:
- Feature driven – When a particular feature is being evaluated
- Performance driven – When an algorithm is being tested
- KP driven – KPIs of the entire system are identified and analysed.
Vision for Synthetic Data and Virtual Simulation Tool
While it already has unique products delivering key solutions for exponential ADAS/AD development, the company is geared up for completing the loop of products by launching its own tool for generation of synthetic data and virtual simulation & validation.
The vision for this product is categorized in four ways as shown in Figure 4 –
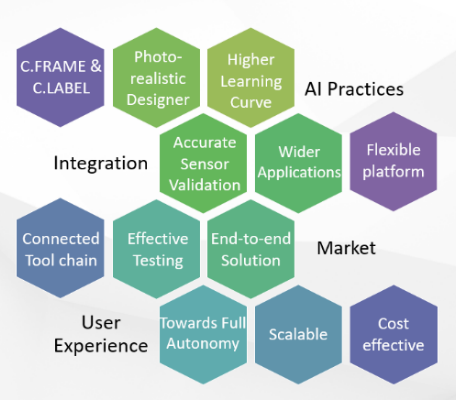
Integration
C.FRAME and C.LABEL are extensible tools developed by CMORE/EC.Mobility which are already competently providing solutions for data recording, data visualization and data labelling. The synthetic data tool will integrate the highly efficient framework of C.FRAME & C.LABEL with photorealistic virtual environment designer, seamless virtual simulation, sensor validation and effective testing to deliver a complete connected tool chain.
Artificial Intelligence Practice
The tool will increase productivity of AI practices by giving a higher learning curve, wider applications and a flexible platform. With high emphasis on Model-in-loop and Software-in-loop frameworks, the tool will provide a reliable testbed for ADAS/AD algorithms.
User Experience
The integration of highly productive tools and technology will allow user to drive towards full autonomy with a flexible framework and connected tool chain leading to faster ADAS/AD development.
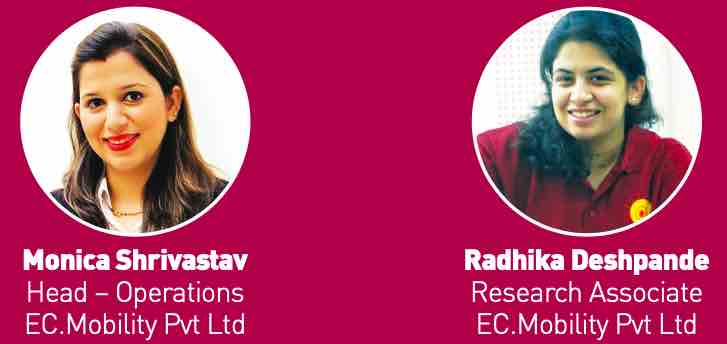
Authors: Radhika Deshpande, Monica Patil
EC.Mobility Pvt Ltd http://www.ec-mobility.biz
Published in Telematics Wire