Autonomous Vehicles – A Waymo Perspective
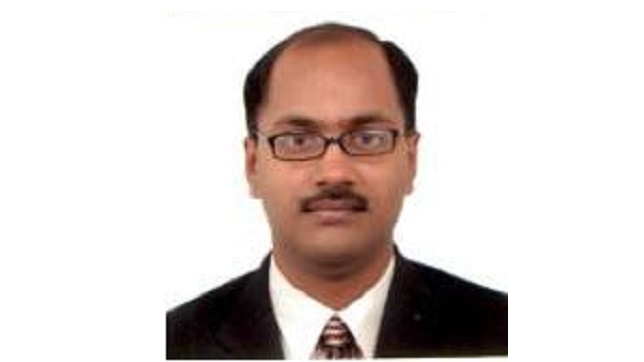
Autonomous driving technology is a promising, rapidly evolving, disruptive game-changer that will not just change our societal structure but earmark a radical evolutionary step beyond just commute. The introduction of autonomous vehicles (AVs) on public roads is perceived to be a futuristic change in the way human transportation is conceived and conducted. But what is more intriguing is the epoch of significant transition where a vehicle’s control is with an onboard computer system while the occupants enjoy their travel time, sublimating to the technology’s prowess of handling imperative artefacts like safety and seamless navigation on public roads. The nuanced concern about any technology, however, is building trust among the optimistic technology adopters through culture-changing technical innovation.
The primary conduit of human mobility has undoubtedly evolved and expanded its paradigm from being at the realm of the human right to resonating with the quality of life of the masses. Industry proponents believe that the world is ready to embrace autonomous vehicles in the foreseeable future with the reincarnation of AVs to solve the deep-seated problems of the general populace. Some of the proximal problems that we now face are with road-related safety, travel time efficiency, and repurposing transportation access for the disadvantaged social groups. Automation changes the fundamental nature of the remaining human work and to sculpt the coming forms of transportation right from attend an event or office without the hassles of driving, dropping kids to school, and provide mobility to those who cannot physically drive, such as the disabled, frail elderly, and children. Thus, AVs can relieve humans of all momentary tactical control and eventually strategic control over mundane tasks.
A lot of ink and ire has gone into campaigning AVs to mitigate the ongoing problem of road traffic injuries and deaths. In such circumstances, AVs could significantly be beneficial in cities grappling with high number of road-related fatalities and accidents, with concomitant reductions in human suffering and expenses for medical treatment and repairs, which is pegged to be around $500 billion every year in the US. If we put our attention to the number of accidents in the year 2016 worldwide, 1.35 million people died in car crashes. Statistics also that around 2.4 million people were seriously injured in 2015 in car accidents. According to Waymo Report, most accidents happened due to human errors, and about 94% of crashes in the US were attributed to human choices and errors.
Google started lead the charge on self-driving cars in 2009. Today, Waymo has taken the baton and is making ground-breaking advances in fully self-driven cars. Waymo’s cars use varied technologies to understand the world around them and get the rider from the starting point to end destination. These cars are equipped with Lidar that sends out millions of laser beams every second to build a detailed impression of the ‘perceptual’ world around it. Additionally, radars are used to detect the distance and the speed of objects in the vicinity. With the high-resolution cameras deployed on the vehicle, it can get visual information such as color of the traffic signal, images of objects around it, etc. precisely up to 300 meters. The vehicle has an advanced onboard computers that imputes and computes the data to understand the world around them. In fraction of a millisecond, these cars perceives the surrounding and performs a predictive analysis to anticipate the movements of the nearby objects.
Constant assimilation of information tranches adds to our experience in life. Similarly, self-driven cars are currently undergoing test-runs for millions of kilometres on complicated city streets or highways to continuously upgrade them with the nuances and subtleties of actual driving conditions and improvising on the safety aspects of pedestrians and vehicles around it. With the ingress of the huge influx of information, AVs pave the way for a safe, radically improved mobility medium.
While testing AVs on the road, information related to different driving patterns are captured and stored. The enormous amount of experience gained through driving across different conditions helps the car learn all the necessary lessons. AVs always look in all directions and spot moving objects approaching towards the car. The estimated position and velocity help the cars predict the next move of the object in a fraction of a second. Night driving conditions are stressful for human drivers, but these cars easily drive in night conditions. The lasers and the radars see where the obstructions are and even observe people not obeying traffic rules, for instance the red-light runner. In such situations, the cars check in all directions all at once and wait if it appears to be a red-light runner. Even in the storms a pedestrian may not be visible by naked eye. These cars see things well in advance. If someone walks across the road, these cars sense the movement using its lasers and radars, and slow down. All this happens in a millionth of a second. A usual person has a lot of built-in assumptions on how the world works. However, the car is always thinking and is designed to consider the worst. We might not be 100% alert while driving as you may get sleepy, drowsy, drunk, distracted by phone calls or by the children in the back seat. The car always pays attention to all of these factors and tries to think ahead. The cars do not just understand the world around but can make those predictions in the blink of an eye for you before you could really understand exactly what is going on.
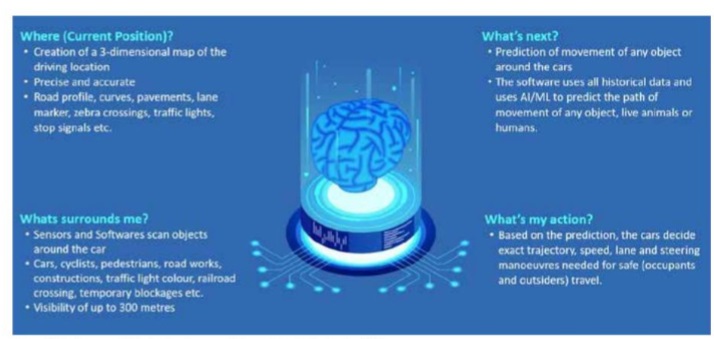
Industry trends
Although technology heavyweights and early-stage start-ups are angling to get a slice of the self-driving pie, Google-Waymo, being the front-runners of this technology, is in the driver’s seat with its largest collective experience garnered over the years. In July this year, AutoX, backed by Dongfeng, SAIC and Alibaba, became the second firm after Waymo to test autonomous cars on the California roads in the presence of a safety driver. WeRide and Baidu have been testing their autonomous vehicles in China but use a remote control to take control of their vehicles in adverse conditions. AutoX, however does not have a remote centre, as it believes that use of remote control would lead to safety issues due to stability and cyber treat issues linked to 5G signals. In China, Pony.ai, a Toyota-backed venture, and Didi Chuxing have been testing their autonomous cars with one or two safety personnel deployed in the vehicle to take control in case of unexpected situations. Keeping in view of the above, Waymo’s AVs are completely autonomous, as it is built on Waymo’s 3-layer technology, which is narrated in subsequent sections of the article.
Waymo’s 3-layer technology as mentioned in their white paper Safety Methodologies and Safety Readiness Determinations, October 2020 has been elucidated below:
Figure 2 A brief and simple explanation of the three layers is given below.
The Hardware Layer
The hardware layer of AVs comprises four major subsystems: base vehicle platform, motion control actuators, attached suite of sensors, and computational platform to run advanced software. Each of these subsystems is important from both safety and performance standpoints of AVs.
Base Vehicle
Waymo’s base vehicle platform is in line with the physical safety of passengers. Its current fleet is comprised of Chrysler Pacifica Hybrid minivans from FCA, which have a 5-star safety rating from NHTSA.
Motion Control
As a pre-requisite for AVs, redundant braking and steering actuators are considered necessary for safety and complete control in case of a system malfunction. In human-driven vehicles, human drivers have the decision-making ability to physically steer or stop the vehicle in case of system failures with or without the help of driver assist features. However, in driverless operation, the Automated Driving System (ADS) is deployed to detect faults in every component of the steering or braking system and also ensure control of the vehicle.
Sensing
Apart from the base vehicle and motion control systems, Waymo has added a host of sensing systems, including Lidar, Radar, cameras, inertial and audio units, which provide an expansive understanding of the driving environment as well as the precise location of the vehicle at any given point. Like the motion control system, these sensing systems are designed to meet the rigorous performance and safety norms.
Computational Platform
Waymo has developed a state-of-the-art computational platform that combines extreme performances with proven reliability and fault tolerance. Its advanced artificial intelligence in ADS can cater to the most critical computational demands of the driverless car. In addition to the advanced performance, they have equipped their computer system to execute the appropriate amount of redundancy. In case of the main computer malfunctions, an additional computer system is activated to control and stop the vehicle safely. In addition, Waymo has developed and tested multiple design choices such as redundant power systems to failproof the secondary computer system.
Fault Detection and Response
In the earlier part of the article, we briefly mentioned about the relevant fault tolerance requirements and the associated verification. In the subsequent sections, we have elaborated on the behavioural layer and the strategies to address detection and response to software faults. By definition, the Level 4 ADS should achieve a minimal risk condition without human intervention in the event of a failure of either the ADS or another vehicle system that interferes with the ADS to perform the dynamic driving task or while encountering conditions beyond the scope of the approved Operational Design Domains (ODD)’s specifications. In addition to the fault detection and fault tolerance mentioned in the sections above, the system has a predefined portfolio of appropriate fault responses to any failure. The proper response will vary according to the type and extent of the system failure and the surrounding traffic situation.
Cybersecurity
While fault tolerance is a critical indicator to gauge the robustness of a system against internal failures, cybersecurity is the other aspect of the system’s resilience to avoid, detect, and respond to external attacks. It is evident in the modern world that attractive targets are frequently attacked; hence, sound security engineering practices are required to ensure robustness of the system and significantly mitigate cybersecurity risks. Although stated in the hardware section, the process extends beyond the software and operations layers. Waymo has built its security framework based on the NHTSA cybersecurity guidelines.
The Behavioural Layer
The behavioural layer ensures that the software is capable of executing safe, driverless manoeuvring of AVs on public roads. Unlike the hardware layer, where more traditional, deterministic safety methodologies can be readily used to measure safety, the behavioural layer requires solving for an infinitely variable set of inputs like actions of other objects on the road and roadway conditions. The complexity of the challenge requires the use of sophisticated algorithms and specialized evaluation methodologies to determine the performance of these algorithms. There are three primary capabilities for evaluating the performance of the ADS’ behavioural layer; viz. avoidance of crashes, completion of trips in driverless mode, and adherence to applicable driving rules.
Hazard Analysis
Waymo leverages hazard analysis techniques to develop and test safety-critical software that adheres to the established industry best practices. Hazard analysis is a prominent methodology used to identify potential causes of safety risks and ways to either eliminate or mitigate these hazards early in the development phases. In the last few decades, reliability and safety professionals were considered as the hazard analysis techniques using deductive methods such as Fault Tree Analysis (FTA) and System-theoretic Process Analysis (STPA), and inductive methods such as Failure Mode and Effects Analysis (FMEA).
Scenario-based Verification Programs
Waymo uses a plethora of scenario-based testing approaches to ensure ADS’ capability of basic behavioural competencies as well as advanced functionalities. The competencies and functionalities that require evaluation in these test programs are derived from systematic methods of describing competencies needed for naturalistic driving research data and public road testing (real world conditions). The virtual test scenarios are either mined from public road driving logs, procured from closed course driving logs, or developed from scratch in simulation-only workspaces. Additionally, Waymo selectively conducts physical tests on candidate software on a closed course. These scenario-based test sets are used to evaluate ADS’ performance across broad spectrum of conditions. One such example of a testing program is the ‘Collision Avoidance Testing Program’. In addition to demonstrating an AV’s capabilities in “normal” driving situations as well as in system failure conditions, an ADS, within reason, should have some capability to avoid or mitigate crashes in urgent situations relevant to the ODD and caused by the behaviour of other road users. It has seeded this test program with scenarios based on the naturalistic driving data and crash databases.
Simulated Deployments
With test processes and structured development, one can effectively establish the evidence for a performant system. It is crucial to undertake additional measures to validate the methods used to this point as well as objectively evaluate the ADS against critical performance indicators. To achieve these goals, Wayno consistently put its system through simulated deployments, wherein its strives to answer the fundamental question, “if this system was deployed in driverless mode, how would it perform?”
These simulated deployments can occur in two main methodological variants. The first variant is one that is executed by re-simulating a certain software version and system configuration against historical logs. This method has its own advantage of being highly scalable, allowing for extreme acceleration of results, and allows individual developers to execute the evaluation themselves. To ensure the credibility of simulations, Waymo has an ongoing simulation credibility process.
The second variant involves driving on public roads with the added supervision of a vehicle operator, and only simulating the points in time where the operator took control of the vehicle, and is usually known as public roads driving with counterfactual simulations. This approach has the advantage of having extreme credibility, since the software version under evaluation was controlling the vehicle in real time for most of the time.
By leveraging both simulation and real-world driving scenarios, Waymo can achieve a level of scale not possible in real driving alone as well as a level of credibility not possible in simulation alone. In both methods, three critical parameters such as avoidance of crashes, completion of driverless trips, and adherence to rules of the road are critically analyzed.
The Operations Layer
Fleet Operations
The application of Waymo’s safety methodologies at the hardware and behavioural layers produces highly capable AVs. The operating a fleet of Waymo’s AVs on public roads requires additional methodologies to manage risks that may arise during these operations as well as derive data that can be fed into the hardware and behavioural layers to ensure continuous improvement. In numerous ways, these final processes serve as the final layer of validation, ensuring that the product and the requirements adhere to the needs of the customers.
Waymo’s safety program ensures application of industry-leading safety practices for operating AVs, which include fatigue management program for our trained vehicle operators and coordination with law enforcement agencies and emergency responders to deal safely with driverless vehicles. Emergency responders plan and prepare extensively to ensure that incident response capabilities are ready to be addressed for a range of events. Moreover, passengers have immediate voice access to the support team for ready assistance seamlessly. Waymo has a fleet response team that can provide remote assistance to the ADS in adverse scenarios. Waymo’s ADS is designed to recognize unexpected situations and contact its fleet response team for additional data on ADS’ visual feed and provide additional contextual information.
Risk Management and the Field Safety Process
Despite the thoroughness of Waymo’s safety methodologies discussed above, a complete safety program must address the residual risks that may remain from these processes and new risks revealed during actual operations. Waymo’s safety program encompasses a full life cycle of AVs. Waymo’s Risk Management Program (“RMP”) identifies, prioritizes, and drives the resolution of potential safety issues before the new or updated features or software are used on public roads or tested at the structured test facility. Issues are prioritized based on a consistent framework for categorizing the residual risk and escalated according to the category, ensuring priority resolution to highest risks and appropriate level of review.
Safety Governance
An effective safety program should include a governance process for making important safety decisions based on the outputs of various safety methodologies. Waymo’s governance process includes a tiered system of analyzing safety issues that arise from field safety.
Levels of Autonomous Vehicles
Autonomous Vehicle Technologies
A schematic illustration of the hardware components of an autonomous car is given below for a complete understanding of such vehicles. This was released by University of Michigan.
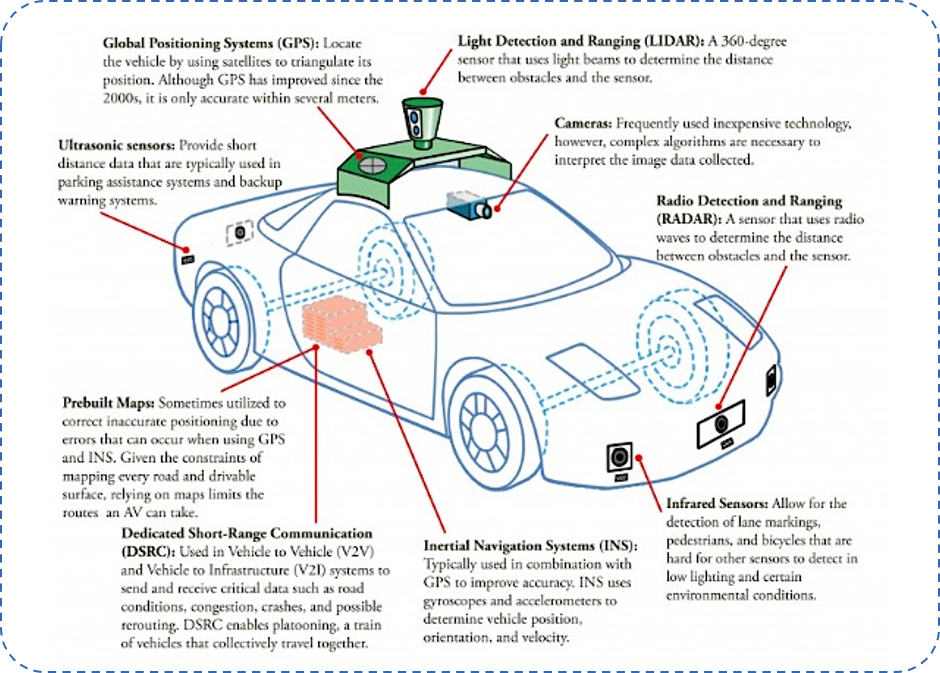
Although AV technology is at a progressive stage, it represents a fast-paced field of modern technology, as companies compete for dominance in this important field of emerging transportation capacity. AVs will extend its profound effects within the automotive sector to not just cars but also trucks, long distance trailers, tractors and two wheelers. AVs possess much more intelligence and operational capacity than yesteryears and will lead to the emergence of an integrated transportation infrastructure.
Source:
https://www.reuters.com/article/us-autox-autonomous-idUSKBN28D047
DOT and NHTSA, Federal Automated Vehicles Policy, September 2016, p. 9, https://www.transportation.gov/AV/federal-automated-vehicles-policy-september-2016
https://www.everycrsreport.com/reports/R44940.html
Author:
Anupam is an United Nations speaker on Electric Mobility, a tech evangelist and a passionate business professional with global experience in consulting, strategy, innovation, key account management, & product management in diversified industries. He worked in Tata Motors, Mahindra & Mahindra, Wipro Technologies and Tata Technologies. He managed the relationship with the largest automotive clients in India, Japan, NA, Germany and France. He is currently pursuing PhD on EV branding for early adoption at IIM Ranchi.
Published in Telematics Wire