Real autonomy in the autonomous vehicle: A Neuroscience-computing approach
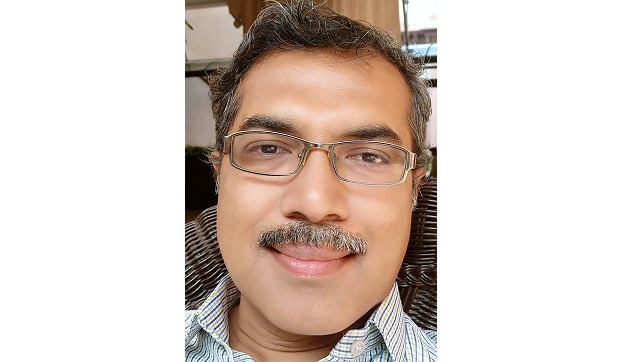
The future of transportation braces the high level of automation and connectivity defined by software. An Autonomous vehicle (AV) will be a key enabler shaping driving automation. When successful with full automation and networking, AV allows passengers to travel more comfortably and safely with a new mobility paradigm. To capture the AV space many auto giants and technology companies are aggressively pushing the limits of science and technology to reach customers asserting the potential to gain an economic and social foothold. In the next decade, stakeholders are set to explore new frontiers to achieve human-like remarkable results in driving automation. SAE Level 2 to Level 3 is the current focus for automated-driving systems. Moving from SAE Level 3 to Level 4 and Level 5 (Human-Out-Of-The-Loop) is all on the continuum.
Driving without a human driver behind the wheels looks fascinating but Intel’s study reveals that many consumers don’t feel safe using AVs with current technology. But on the contrary, nearly 63 % of the U.S population will be driving fully automated vehicles by the year 2070.
Trust, safety, and security are three pillars on every OEM’s and tech companies mind responsible for creating the brave new world of AVs. These three entities describe risks along with opportunities in making AVs accepted by the general public. True to its value comes with greater challenges.
To date, the AV industry is less confident to drive in real public driving scenarios with flawless operation irrespective of road condition, visibility, traffic density, and weather conditions due to sensor and algorithmic limitations. The fatal accident in recent times operated by Tesla and Uber self-driving is an admonishing reminder.
In contrast, in most cases, human drivers are not only known to drive comfortably and safely in a familiar environment but can also handle complex, fast-changing, and partially-observable unknown environments with ease. The reason for being successful is humans depend upon their knowledge gained through unsupervised and supervised exploration for experiencing the diversity of real-world settings and reuse the learned concepts and abstractions built from during lifetime driving to quickly adapt from only a few instances of evidence. This is one of the holy grails that engineers and researchers strive to develop and demonstrate in safety-critical applications like self-driving today. There is a lot of hype surrounding AVs. The reality is many automakers concedes highly-automated-driving (HAD) will take much more time than anticipated.
The current paradigm of AV functionality consists of:
1.AV Sensor suite: For the AV to handle complex driving scenarios, information from the camera, lidar, radar, etc., must be intelligently connected as shown in figure 3 and brought into context. Different sensors are required to perceive the environment and unequivocally comprehend digitally every driving scene in less favorable environmental conditions. AV sensor suite powered by sensor fusion provides a sweeping and 360-degree view of the driving condition. AVs will be as good as its sensor suite and algorithm running it.
2.Data-driven approach: The widely adopted approach is the data-driven computing technique consisting of four modules like perception, localization & mapping, planning, and motion controls as depicted in figure 4.
In this paradigm, the information is processed and computed serially. The environment perception data is obtained by sensors suite which are used in localization, manoeuver decision, path, and speed profiling to derive motion controls. Driving tasks are accomplished either using a static rule-based engine or Artificial Intelligence (AI) techniques. Artificial neural network (ANN) is widely used to tackle the challenges like object detection of driving automation is called ‘Narrow AI’ as shown in the below figure 5 and 6.
However, the data-driven approach fails to address unfamiliar scenarios and hence poor in adaptability and self-learning. The current state-of-the-art ANN is quite not ready to be used in safety-critical applications like self-driving cars as it encounters a fast-changing world filled with noisy stimuli signals and detect unpredictable changes during its journey. It is being widely used as a ‘magic wand’ without knowing its limitations, background, and less emphasis on understanding the DNA of cognitive science underpinning them. Most of the ANN model works well with the simulation-based approach but fails when operated using hardware to conceptualize and generalize in the real world-a phenomenon called ‘reality gap’. Moreover, ISO 26262 does not mention guidelines using AI.
To mitigate the challenges of SAE Level 4 and above we can take a cue from current developments in neuroscience. The next big wave of Artificial General Intelligence (AGI) with human-like cognitive is the long-term goal of AI is dogged from the cognitive neurobiology domain to address the current problems in the AV industry. Some of the concepts described below show promise and can be applied to make AVs more robust, safe, and, could reach nearer to an average human driver in terms of performance. The next section describes a few concepts stemmed from neuroscience:
1.Learning algorithms: The non-biological aspect of the majority of deep learning techniques applied in AV at present is the supervised training process with backpropagation algorithm that suffers from two problems: requiring a massive amount of labeled data and a non-local learning rule for changing synapse strength. A biologically plausible plasticity learning algorithm is comparable with standard backpropagation based ANN models minus two problems aforementioned. These algorithms banks upon an unsupervised way of learning and utilizes local learning rules leading to good performance and generalization on the benchmark datasets. Taking a cue from neuroscience development, few players investigating and building a robust lightweight platform based on signatures, bottom-up, fine grain, unsupervised learning enabling a comprehensive understanding of AV surroundings.
2.Episodic memory: To mitigate the challenges thrown by the real-world, Autonomous vehicle companies have started using the idea of world models and constructive episodic simulation hypothesis (CESH) in planning and imagination of future events remembering from past experience. AVs moving in complex and constrained noisy environments can leverage upon the idea of a hippocampal episodic memory controller that can guide decisions based on a single past episode that it remembers or even when it needs to transfer from one task to another as reflected in Rapid & Zero/Single-shot learning.
3,Cognitive model: A novel self-driving framework combined with an attention mechanism inspired by the human brain has been reported. The framework the brain-inspired cognitive model with attention (CMA) simulating the human visual cortex, LSTM, and cognitive map for attention mechanism. It can handle the spatial-temporal relationships, to implement the basic self-driving missions.
4.Metacognition: To deal with surprising events metacognitive learning has been used in AI agents to increase the level of autonomy and has the potential that would compensate for the weakness of model-based reinforcement learning (overconfident algorithm). Again it has roots in decision neuroscience. Metacognition refers to an ability to evaluate an agent’s own thought process, such as perception, valuation, inference, and to report the level of confidence about choice leading to an outcome. This approach resolves exploration-exploitation tradeoffs and brings a performance boost to decision-making models in AV.
5.Virtual brain analytics: Self-driving vehicles implicating current ANN models are considered as ‘black boxes’ which makes it harder to detect the faults and where the impossibility of understanding leads to serious safety issues related to validating the computations of critical components. Efforts in the direction of decoding black box to grey box (if not white) are shown but proving the faithfulness of the automatically generated explanations on neural network models is the greatest challenge as of now. However, recent techniques like lesion and neuroimaging can be applied to get deeper insights to computation of AI systems. It also increases the interpretability of these systems so as to improve the safety issues.
6.Decision-making model: In a normative real-world, decision making in mammals is central to cognition and ubiquitous in our daily life. A decision is a process that weighs priors, evidence, and value to generate a commitment to a categorical proposition intended to achieve a particular goal. The recent development in neuroscience imaging proves the dynamism in the mammalian brain circuit during the decision process. The brain is made up of more than 100 trillion neurons and synapses making a complex network to learn, but it takes just an image to extract the knowledge and builds a generalized conception about the overall environment quickly in an unsupervised way. The cortico-basal-ganglia-thalamo–cortical loop (CBGTC), a neural substrate, is involved collectively in the decision-making process and recent evidence in decision neuroscience has shown that multiple regions of the brain networks are associated with specific facets of decision making. The different neural circuit gets implicated with a decision made under-risk and uncertainty, probabilistic reasoning, value, and reward-based. Evidence accumulation based algorithm like multi-hypothesis test can be proposed for the arbitration process in case of a conflict between multiple decision-making systems in AV.
David Cox, MIT-IBM Watson chief, mention that days are not far when the brainpower of rat if properly applied is sufficient to drive a car. Live brain uploading or brain digitizing is the new frontier of research and going to squeeze the biological brain more to fill the gaps and upload in new AI.
To conclude, to be successful the system on its own has to perform well and meet the desired expectations of the goal in both normal and adverse situations. The above-highlighted areas of neuroscience have the potential to lend more real autonomy to make the autonomous car self-learned and be adaptive. Historically, the flow of information from neuroscience to AI is reciprocal. It’s not a compulsion neither to apply biological plausible concepts or a mandatory requirement but a seeing through the lens from neuroscience springs new algorithms, architecture and validation of current AI techniques. The principles of neuroscience embody could inspire the development of comparable strategies that confer flexibility and efficiency in self-driving cars by filling the gaps that exist in ANN. The need of the hour is to have an inter-disciplinary approach from Computer science, Neuroscience, and Cognitive psychology as well as hardware support to convert the science-fiction theme to reality.
Author:
Sham Sundar Rao is a Technical Lead in Conigital-ICAV Tech. Has vast experience in SAE Level 4 automated driving systems mainly in algorithm development, artificial intelligence, sensor networks, and automotive safety. He studied at Politecnico di Torino, Italy for a Master’s degree.
Published in Telematics Wire