AV – Insights into Development and Challenges
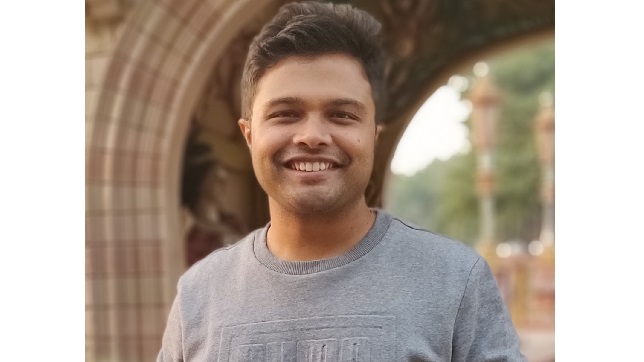
How would you like it if your favorite car can drive you to your office daily without your intervention? Or if your car can drive you to your favorite restaurant while you spend leisure time with your family in the car? Have you ever thought about how we can make driving safer?
If this excites you then there is some good news! The automotive world is in a race against time to make Autonomous Vehicles (AV) a reality. These vehicles are expected to make mobility more convenient and safe. However today only partially autonomous cars and trucks with varying amounts of self-automation exist.
Movement of people and goods have always been a fundamental part of our lives since ages. Due to this, mobility has been a very important aspect to mankind and there have been numerous efforts to enhance the same. Since the time the wheels were invented, the way we move around has drastically changed. Today we are in a pursuit to enable mobility with more independence and intelligence in order to make it smart, convenient, safe and green.
So what is Autonomous Driving?
Autonomous driving can be understood as handing over the vehicle guidance function and authority to a vehicle.The transfer of the guidance function may be for a limited location or a limited time and can potentially be interrupted by the driver. Basically, the autonomous vehicle is capable, without human assistance, of deciding the route, lane, and interventions in the driving dynamics like braking or steering. Such a function is subject to both technical and social requirements. Implicitly an autonomous vehicle must pose no greater danger than a vehicle controlled by a person!
The level of autonomy of a self-driving car refers to how much of the driving is done by a computer versus a human. The higher the level, the more of the driving that is done by a computer. SAE International (Society of Automotive Engineers) provides taxonomy and definitions for different levels of Driving Automation. Six levels are defined starting with Level 0 (No driving automation) up to Level 5 (Full driving automation).
Self-driving cars can be further distinguished as being connected or not, indicating whether they can communicate with other vehicles and/or infrastructure, such as next generation traffic lights. Most prototypes do not currently have this capability.
Design considerations for development of AVs
Though the design details for autonomous vehicles widely vary, one way to equip the vehicles with intelligence is to mimic the capabilities of human information processing itself on the vehicle through reliable models. This involves capturing the real world data through sensors (Sensing), transforming the data into a cognitive representation (Perceive) and Interpretation of the information through which decisions can be taken on the reaction (Stimulus). It is necessary to perform this cycle continuously with the highest precision by overcoming the timing and hardware resource constraints. In addition interior or driver monitoring further enhances safety by detecting driver drowsiness or distraction.
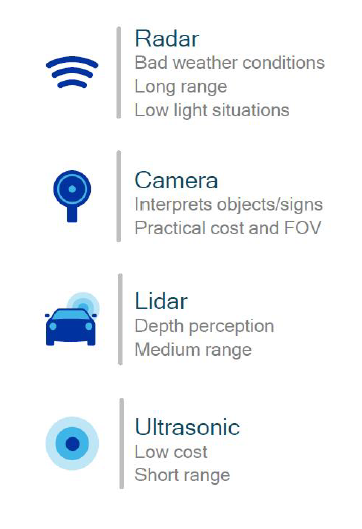
Capturing of real world data includes all processes relating to the discovery and recognition of relevant information. During driving the most significant inputs are the visual, acoustic and tactile information that are realized through sensory organs of eyes, ears and skin receptors respectively. This cooperative sensing is realized with use of environmental sensors and technologies like RADAR, Video, Lidar, GPS and V2X (Vehicle to Everything. See picture below). Equally important is to capture the information of the vehicle under test. This is accomplished by continuously reading information from vehicle sensors like wheel speed, yaw-rate or steering wheel angles sensors.
So far, the Internet has played an only marginal role in vehicles. Up until now, the use of data links has been restricted mainly to infotainment and navigation support. For the development of autonomous vehicles, the internet is believed to bring in more capabilities. For example the Vehicles equipped with sensors and V2V communication devices could expand their horizon via cooperative sensing using internet and data cloud. But for this a reliable network with high quality of service and data integrity becomes necessary.
Though the environmental sensors like Radar, Video or Lidar are capable to an large extent in collecting detailed data of a car’s surrounding environment, there is lot of room for enhancing system cognition and situational awareness. Transforming the sensor data into a meaningful representation of a real world involves exhaustive cognitive processing. Artificial Intelligence related techniques have made it possible to push the boundaries that were imperative in the conventional models. Large neural networks are trained for tasks like Image Classification, Object Detection and Classification, Road Sign and Lane Recognition to name a few.
So instead of manually writing a software function for an autonomous vehicle to follow, such as , “stop when you see red light”, deep neural networks enable autonomous vehicles to learn how to navigate the world on their own using sensor data. These algorithms are inspired by human brain, implying they learn by experience. However the Deep Learning based techniques for computer vision are dependent on training data, a lot of which is being already collected and processed. Hence through deep learning, vehicles will “learn” to understand its environment.
One of the foremost tasks involves synchronizing the data from various sensors since it is likely the data is captured at different time instances. The primary scope of the perception would be to provide answers to questions like what do we see or how do the objects look like. To get a complete and accurate perception of the surroundings it would be necessary to fuse the data from multiple sensors. Combining the information from different sensors helps to leverage the benefits of different sensor technologies. The primary objective of data fusion is to merge the data from individual sensors so as to combine their strengths in a beneficial way and reduce individual weaknesses. For example, RADAR provides reasonably accurate measurements of object longitudinal distance and velocity in various weather conditions. However these sensors have limitations in measuring lateral distances, reading road signs and lanes. Such gaps can be offset by utilizing information provided by the Video. This makes it possible to reduce false interpretations and improve accuracy and improve the system’s ability to detect objects more robustly.
The ability to interpret and predict the current traffic situation is essential for being able to automatically derive reasonable decisions. Though several models exist which satisfy the requirements for lower levels of autonomous driving, more needs to be done for development of methods and algorithms to acquire better situational awareness at a safety-relevant integrity level. These involve Intention and Behavior models to predict behavior of driver and other traffic participants.
For higher levels of autonomous driving Path Planning plays a key role in navigating the vehicle safely to its target destination by avoiding obstacles. Simultaneous Localization and Mapping (SLAM) is a common technique used in autonomous navigation where a vehicle builds a global map of current environment and uses this map to navigate or know its location at any point of time.
Next the decision on reaction is conveyed to vehicle motion control modules that drive the necessary actuators to realize required maneuvers like lane change or acceleration or braking.
Challenges and Conclusion
Nevertheless autonomous vehicles encounter several challenges. If autonomous driving is intended to improve road safety by the widespread use of vehicles with such capabilities, the safety objective must be measured against the status quo of road safety. The available testing methods needs to be improved to validate such complex systems. Concepts for the assessment of human and machine driving performance are required. Hence newer metrics may have to be defined to assess the performance. This could be a greater challenge than the development of artificial intelligence for autonomous driving itself.
Secondly, technological development requires large investments that can only be redeemed by an appropriate market demand. If the market does not accept the developed product, financing further developmental steps may prove difficult.
Next the autonomous vehicles would need a strong infrastructural support. For example in terms of road topology or lane markings. Collective traffic control is dependent on technologies like V2X which needs further development towards a level of maturity that allows usage in on road vehicles.
Finally introduction of such cars in the market needs to satisfy legislation requirements of various markets as well as legal requirements (Who’s liable in the case of an accident?). Though it is clear that the automated systems on one hand can increase convenience and road safety it is likely to bring in some automation related risks to road traffic that is not fully known. The question of acceptance in future therefore remains significant.
Disclaimer
The views expressed in this article are my own and do not necessarily represent the views of the organization I work with.
Author:
Enthusiast for Autonomous Driving technologies working as a Technical Architect at Robert Bosch Engineering and Business Solutions with over 11 years of Industry Experience. My primary focus is on Perception related topics involving Driver Assistance and Autonomous Driving Systems with expertise in Software Engineering.
Published in Telematics Wire