Deep learning helps detect distracted driving behavior
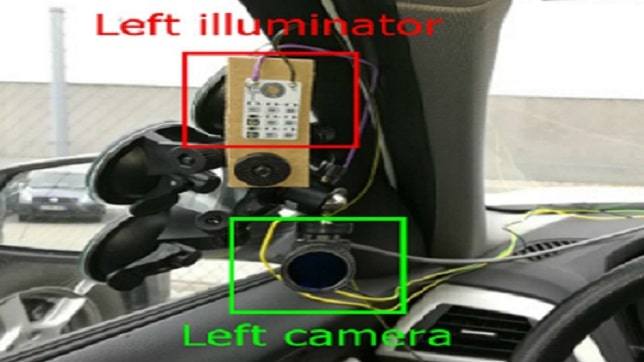
German-based company ARRK Engineering is developing a new advanced driver assistance system (ADAS) that detects sources of driver inattention caused by elements other than drivers falling asleep at the wheel.
ADAS detect t driver’s tiredness via analysis of driving behavior or use camera-based computer vision to monitor head and eye motion to gauge whether a driver is tired. If the driver’s eyes closing entirely and remaining closed represents an almost sure indication. In either case, the ADAS can emit an alert and rouse the driver.
While current vision-based ADAS systems may readily detect the radical changes in a driver’s viewing direction and head position caused by falling asleep, registration of more subtle changes associated with other sources of driver inattention such as eating, drinking, or using a smartphone present a challenge.
ARRK Engineering’s new driver distraction system overcome these shortcomings. The system consists of two FLIR Machine Vision FL3-U3-13Y3M-C cameras with 940 nm infrared (IR) LEDs and 70° field of view attached to the A-pillars on either side of the driver. Both cameras run at 30 fps and capture 8-bit greyscale images at 1280 x 1024 resolution. A Raspberry Pi 3 Model B+ single-board computer synchronizes image capture by sending a signal to both cameras.
Each camera features a Midwest Optical Systems LP780 IR long-pass filter that blocks out most light under 780 nm wavelengths, to make sure light is captured primarily from the IR LEDs, versus capturing ambient light. Blocking visible daylight also eliminates shadow effects in the driver’s immediate area that may interfere with the accuracy of face recognition algorithms.
To generate images for training and testing, 16 subjects of varying gender and age pretend to drive a stationary vehicle and simulate driving behavior like moving the steering wheel, looking at the side and rearview mirrors, looking out of side windows, and paying normal attention to the road.
The ResNeXt-34 and ResNeXt-50 models performed best, with 92.88% accuracy on the left camera and 90.36% accuracy on the right camera. The test results indicate proof of concept for a driver assistance system that recognizes when drivers eat, drink, or use a smartphone, and emit an alert when the driver engages in these hazardous behaviors.
ARRK Engineering plans to further develop the system by analyzing whether the classification of objects, for instance, smartphones and beverages, and determining the relative positions of those objects, or where the driver is holding them, potentially with bounding box detection and semantic segmentation techniques can improve the system’s accuracy.